Clase 13: Introducción al paquete Scipy
El paquete Scipy es una colección de algoritmos y funciones construida sobre Numpy para facilitar cálculos y actividades relacionadas con el trabajo técnico/científico.
Una mirada rápida a Scipy
La ayuda de scipy contiene (con help(scipy)
entre otras cosas)
Contents
--------
SciPy imports all the functions from the NumPy namespace, and in
addition provides:
Subpackages
-----------
Using any of these subpackages requires an explicit import. For example,
``import scipy.cluster``.
::
cluster --- Vector Quantization / Kmeans
fftpack --- Discrete Fourier Transform algorithms
integrate --- Integration routines
interpolate --- Interpolation Tools
io --- Data input and output
linalg --- Linear algebra routines
linalg.blas --- Wrappers to BLAS library
linalg.lapack --- Wrappers to LAPACK library
misc --- Various utilities that don't have
another home.
ndimage --- n-dimensional image package
odr --- Orthogonal Distance Regression
optimize --- Optimization Tools
signal --- Signal Processing Tools
sparse --- Sparse Matrices
sparse.linalg --- Sparse Linear Algebra
sparse.linalg.dsolve --- Linear Solvers
sparse.linalg.dsolve.umfpack --- :Interface to the UMFPACK library:
Conjugate Gradient Method (LOBPCG)
sparse.linalg.eigen --- Sparse Eigenvalue Solvers
sparse.linalg.eigen.lobpcg --- Locally Optimal Block Preconditioned
Conjugate Gradient Method (LOBPCG)
spatial --- Spatial data structures and algorithms
special --- Special functions
stats --- Statistical Functions
Más información puede encontrarse en la documentación oficial de Scipy
import numpy as np
import matplotlib.pyplot as plt
Funciones especiales
En el submódulo scipy.special
están definidas un número de funciones
especiales. Una lista general de las funciones definidas (De cada tipo
hay varias funciones) es:
Airy functions
Elliptic Functions and Integrals
Bessel Functions
Struve Functions
Raw Statistical Functions
Information Theory Functions
Gamma and Related Functions
Error Function and Fresnel Integrals
Legendre Functions
Ellipsoidal Harmonics
Orthogonal polynomials
Hypergeometric Functions
Parabolic Cylinder Functions
Mathieu and Related Functions
Spheroidal Wave Functions
Kelvin Functions
Combinatorics
Other Special Functions
Convenience Functions
from scipy import special
Funciones de Bessel
Las funciones de Bessel son soluciones de la ecuación diferencial:
Para valores enteros de \(\nu\) se trata de una familia de funciones que aparecen como soluciones de problemas de propagación de ondas en problemas con simetría cilíndrica.
np.info(special.jv)
jv(x1, x2, /, out=None, *, where=True, casting='same_kind', order='K', dtype=None, subok=True[, signature, extobj]) jv(v, z, out=None) Bessel function of the first kind of real order and complex argument. Parameters ---------- v : array_like Order (float). z : array_like Argument (float or complex). out : ndarray, optional Optional output array for the function values Returns ------- J : scalar or ndarray Value of the Bessel function, \(J_v(z)\). See also -------- jve : \(J_v\) with leading exponential behavior stripped off. spherical_jn : spherical Bessel functions. j0 : faster version of this function for order 0. j1 : faster version of this function for order 1. Notes ----- For positive v values, the computation is carried out using the AMOS [1]_ zbesj routine, which exploits the connection to the modified Bessel function \(I_v\), .. math:: J_v(z) = exp(vpiimath/2) I_v(-imath z)qquad (Im z > 0) J_v(z) = exp(-vpiimath/2) I_v(imath z)qquad (Im z < 0) For negative v values the formula, .. math:: J_{-v}(z) = J_v(z) cos(pi v) - Y_v(z) sin(pi v) is used, where \(Y_v(z)\) is the Bessel function of the second kind, computed using the AMOS routine zbesy. Note that the second term is exactly zero for integer v; to improve accuracy the second term is explicitly omitted for v values such that v = floor(v). Not to be confused with the spherical Bessel functions (see spherical_jn). References ---------- .. [1] Donald E. Amos, "AMOS, A Portable Package for Bessel Functions of a Complex Argument and Nonnegative Order", http://netlib.org/amos/ Examples -------- Evaluate the function of order 0 at one point. >>> from scipy.special import jv >>> jv(0, 1.) 0.7651976865579666 Evaluate the function at one point for different orders. >>> jv(0, 1.), jv(1, 1.), jv(1.5, 1.) (0.7651976865579666, 0.44005058574493355, 0.24029783912342725) The evaluation for different orders can be carried out in one call by providing a list or NumPy array as argument for the v parameter: >>> jv([0, 1, 1.5], 1.) array([0.76519769, 0.44005059, 0.24029784]) Evaluate the function at several points for order 0 by providing an array for z. >>> import numpy as np >>> points = np.array([-2., 0., 3.]) >>> jv(0, points) array([ 0.22389078, 1. , -0.26005195]) If z is an array, the order parameter v must be broadcastable to the correct shape if different orders shall be computed in one call. To calculate the orders 0 and 1 for an 1D array: >>> orders = np.array([[0], [1]]) >>> orders.shape (2, 1) >>> jv(orders, points) array([[ 0.22389078, 1. , -0.26005195], [-0.57672481, 0. , 0.33905896]]) Plot the functions of order 0 to 3 from -10 to 10. >>> import matplotlib.pyplot as plt >>> fig, ax = plt.subplots() >>> x = np.linspace(-10., 10., 1000) >>> for i in range(4): ... ax.plot(x, jv(i, x), label=f'$J_{i!r}$') >>> ax.legend() >>> plt.show()
np.info(special.jn_zeros)
jn_zeros(n, nt) Compute zeros of integer-order Bessel functions Jn. Compute nt zeros of the Bessel functions \(J_n(x)\) on the interval \((0, \infty)\). The zeros are returned in ascending order. Note that this interval excludes the zero at \(x = 0\) that exists for \(n > 0\). Parameters ---------- n : int Order of Bessel function nt : int Number of zeros to return Returns ------- ndarray First nt zeros of the Bessel function. See Also -------- jv: Real-order Bessel functions of the first kind jnp_zeros: Zeros of \(Jn'\) References ---------- .. [1] Zhang, Shanjie and Jin, Jianming. "Computation of Special Functions", John Wiley and Sons, 1996, chapter 5. https://people.sc.fsu.edu/~jburkardt/f77_src/special_functions/special_functions.html Examples -------- Compute the first four positive roots of \(J_3\). >>> from scipy.special import jn_zeros >>> jn_zeros(3, 4) array([ 6.3801619 , 9.76102313, 13.01520072, 16.22346616]) Plot \(J_3\) and its first four positive roots. Note that the root located at 0 is not returned by jn_zeros. >>> import numpy as np >>> import matplotlib.pyplot as plt >>> from scipy.special import jn, jn_zeros >>> j3_roots = jn_zeros(3, 4) >>> xmax = 18 >>> xmin = -1 >>> x = np.linspace(xmin, xmax, 500) >>> fig, ax = plt.subplots() >>> ax.plot(x, jn(3, x), label=r'$J_3$') >>> ax.scatter(j3_roots, np.zeros((4, )), s=30, c='r', ... label=r"$J_3$_Zeros", zorder=5) >>> ax.scatter(0, 0, s=30, c='k', ... label=r"Root at 0", zorder=5) >>> ax.hlines(0, 0, xmax, color='k') >>> ax.set_xlim(xmin, xmax) >>> plt.legend() >>> plt.show()
# Ceros de la función de Bessel
# Los tres primeros valores de x en los cuales se anula la función de Bessel de orden 4.
special.jn_zeros(4,3)
array([ 7.58834243, 11.06470949, 14.37253667])
x = np.linspace(0, 16, 50)
for n in range(0,8,2):
p= plt.plot(x, special.jn(n, x), label='$J_{}(x)$'.format(n))
z = special.jn_zeros(n, 6)
z = z[z < 15]
plt.plot(z, np.zeros(z.size), 'o', color= p[0].get_color())
plt.legend(title='Funciones $J_n$ de Bessel', ncol=2);
plt.grid(True)
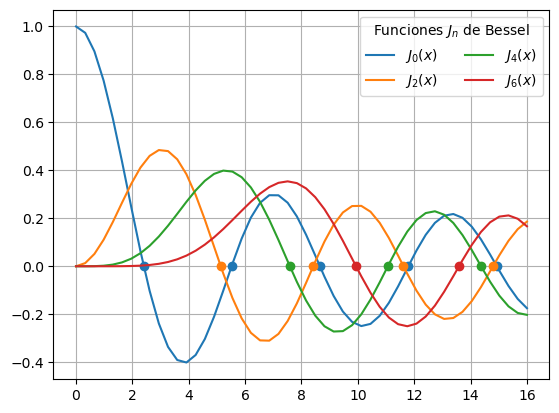
type(p), type(p[0])
(list, matplotlib.lines.Line2D)
# jn es otro nombre para jv
print(special.jn == special.jv)
print(special.jn is special.jv)
True
True
Como vemos, hay funciones para calcular funciones de Bessel. Aquí
mostramos los órdenes enteros pero también se pueden utilizar órdenes
\(\nu\) reales. La lista de funciones de Bessel (puede obtenerse de
la ayuda sobre scipy.special
) es:
Bessel Functions
Zeros of Bessel Functions
Faster versions of common Bessel Functions
Integrals of Bessel Functions
Derivatives of Bessel Functions
Spherical Bessel Functions
Riccati-Bessel Functions
Por ejemplo, podemos calcular las funciones esféricas de Bessel, que aparecen en problemas con simetría esférica:
x = np.linspace(0, 16, 50)
for n in range(0,7,2):
p= plt.plot(x, special.spherical_jn(n, x), label='$j_{}(x)$'.format(n))
plt.legend(title='Funciones esféricas de Bessel $j_n$', ncol=2);
plt.grid(True)
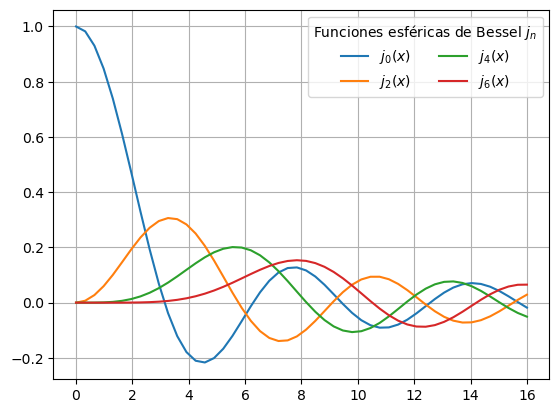
Función Error
La función error es el resultado de integrar una función Gaussiana
mientras que las integrales seno y coseno de Fresnel están definidas por:
x = np.linspace(-3, 3,100)
f = special.fresnel(x)
plt.plot(x, special.erf(x),'-', label=r'$\mathrm{erf}(x)$')
plt.plot(x, f[0],'-', label=r'$\mathrm{ssa}(x)$')
plt.plot(x, f[1],'-', label=r'$\mathrm{csa}(x)$')
plt.xlabel('$x$')
plt.ylabel('$f(x)$')
plt.legend(loc='best')
plt.grid(True)
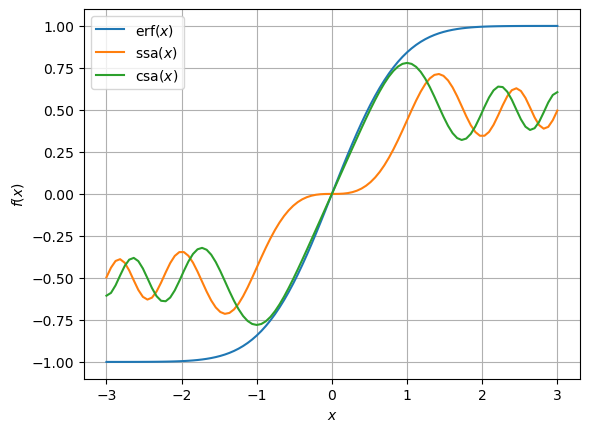
Evaluación de polinomios ortogonales
Scipy.special
tiene funciones para evaluar eficientemente polinomios
ortogonales
Por ejemplo si queremos, evaluar los polinomios de Laguerre, solución de la ecuación diferencial:
x = np.linspace(-1, 1,100)
for n in range(2,6):
plt.plot(x, special.eval_laguerre(n, x),'-', label=r'$n={}$'.format(n))
plt.xlabel('$x$')
plt.ylabel('$f(x)$')
plt.legend(loc='best', ncol=2)
plt.grid(True)
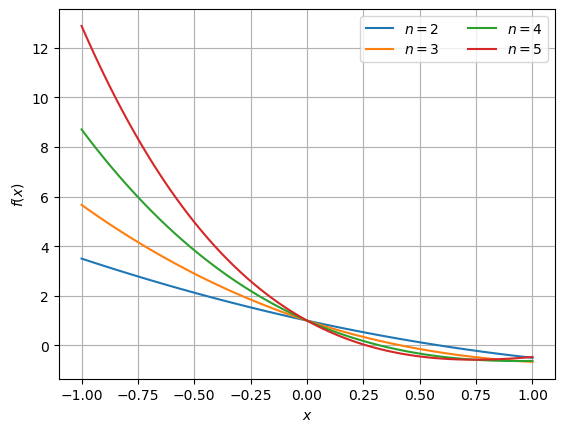
Los polinomios de Chebyshev son solución de
x = np.linspace(-1, 1,100)
for n in range(2,6):
plt.plot(x, special.eval_chebyt(n, x),'-', label=f'$n={n}$')
plt.xlabel('$x$')
plt.ylabel('$f(x)$')
plt.legend(loc='best', ncol=2)
plt.ylim((-1.1,2))
plt.grid(True)
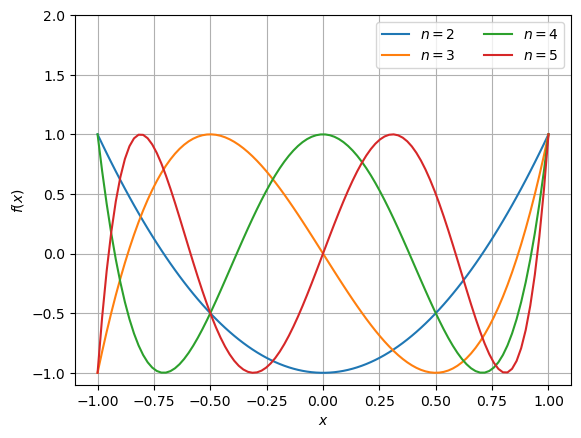
Factorial, permutaciones y combinaciones
Los números factorial (N!) y doble factorial (N!!) pueden calcularse para un valor de N o un array, mediante:
N = np.array([3,6,8])
print(f"{N}! = {special.factorial(N)}")
print(f"{N}!! = {special.factorial2(N)}")
[3 6 8]! = [6.000e+00 7.200e+02 4.032e+04]
[3 6 8]!! = [ 3. 48. 384.]
Hay funciones para calcular varias funciones relacionadas con permutaciones y combinatoria
La función comb()
da el número de maneras de elegir k
de un
total de N
elementos. Sin repeticiones está dada por:
mientras que si cada elemento puede repetirse, la fórmula es:
Por ejemplo, las combinaciones de \(N=3\)
\(\{1,2,3\}\)elementos tomados de a \(k=2\), sin repeticiones,
será comb(3,2)=3
Las combinaciones de \(N=3\) elementos \(\{1,2,3\}\) tomados de
a \(k=2\), con repeticiones, será comb(3,2)=6
N = 5
special.comb(N,3)
10.0
# Si usamos exact=True realiza el cálculo en forma exacta ;)
special.comb(N,3,exact=True)
10
Para realizar el cálculo del número de permutaciones o combinaciones tomados de a distintos valores podemos utilizar un array como argumento \(k\)
k = np.arange(2,4)
special.comb(N, k)
array([10., 10.])
special.comb(N,k, repetition=True)
array([15., 35.])
La función perm()
da el número de maneras de permutar k
elementos de un total de N
elementos. Sin repeticiones está dada
por:
Por ejemplo, las permutaciones de \(N=3\) elementos
\(\{1,2,3\}\) tomados de a \(k=2\) será perm(3,2)=6
y
consiste de las permutaciones:
special.perm(10,k)
array([ 90., 720.])
cuyos valores corresponden a:
Integración numérica
Scipy tiene rutinas para integrar numéricamente funciones o tablas de datos. Por ejemplo para integrar funciones en la forma:
la función más utilizada es quad
, que llama a distintas rutinas del
paquete QUADPACK dependiendo de los argumentos que toma. Entre los
aspectos más notables está la posibilidad de elegir una función de peso
entre un conjunto definido de funciones, y la posibilidad de elegir un
dominio de integración finito o infinito.
from scipy import integrate
x = np.linspace(-10., 10, 100)
def f1(x):
return np.sin(x)*np.exp(-np.square(x+1)/10)
plt.plot(x,f1(x))
[<matplotlib.lines.Line2D at 0x7fb48256c550>]
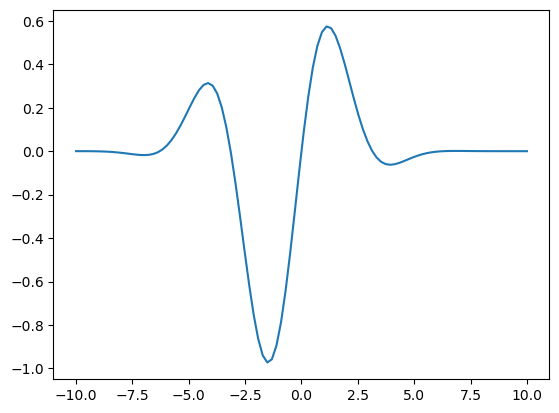
integrate.quad(f1,-10,10)
(-0.3872712191192437, 7.90260497896011e-13)
np.info(integrate.quad)
quad(func, a, b, args=(), full_output=0, epsabs=1.49e-08, epsrel=1.49e-08, limit=50, points=None, weight=None, wvar=None, wopts=None, maxp1=50, limlst=50, complex_func=False) Compute a definite integral. Integrate func from a to b (possibly infinite interval) using a technique from the Fortran library QUADPACK. Parameters ---------- func : {function, scipy.LowLevelCallable} A Python function or method to integrate. If func takes many arguments, it is integrated along the axis corresponding to the first argument. If the user desires improved integration performance, then f may be a scipy.LowLevelCallable with one of the signatures:: double func(double x) double func(double x, void user_data) double func(int n, double *xx) double func(int n, double *xx, void *user_data) The ``user_data`` is the data contained in the `scipy.LowLevelCallable`. In the call forms with ``xx``, ``n`` is the length of the ``xx`` array which contains ``xx[0] == x`` and the rest of the items are numbers contained in the ``args`` argument of quad. In addition, certain ctypes call signatures are supported for backward compatibility, but those should not be used in new code. a : float Lower limit of integration (use -numpy.inf for -infinity). b : float Upper limit of integration (use numpy.inf for +infinity). args : tuple, optional Extra arguments to pass to `func`. full_output : int, optional Non-zero to return a dictionary of integration information. If non-zero, warning messages are also suppressed and the message is appended to the output tuple. complex_func : bool, optional Indicate if the function's (`func`) return type is real (``complex_func=False``: default) or complex (``complex_func=True``). In both cases, the function's argument is real. If full_output is also non-zero, the `infodict`, `message`, and `explain` for the real and complex components are returned in a dictionary with keys "real output" and "imag output". Returns ------- y : float The integral of func from `a` to `b`. abserr : float An estimate of the absolute error in the result. infodict : dict A dictionary containing additional information. message A convergence message. explain Appended only with 'cos' or 'sin' weighting and infinite integration limits, it contains an explanation of the codes in infodict['ierlst'] Other Parameters ---------------- epsabs : float or int, optional Absolute error tolerance. Default is 1.49e-8. `quad` tries to obtain an accuracy of ``abs(i-result) <= max(epsabs, epsrel*abs(i))`` where ``i`` = integral of `func` from `a` to `b`, and ``result`` is the numerical approximation. See `epsrel` below. epsrel : float or int, optional Relative error tolerance. Default is 1.49e-8. If ``epsabs <= 0``, `epsrel` must be greater than both 5e-29 and ``50 * (machine epsilon)``. See `epsabs` above. limit : float or int, optional An upper bound on the number of subintervals used in the adaptive algorithm. points : (sequence of floats,ints), optional A sequence of break points in the bounded integration interval where local difficulties of the integrand may occur (e.g., singularities, discontinuities). The sequence does not have to be sorted. Note that this option cannot be used in conjunction with ``weight``. weight : float or int, optional String indicating weighting function. Full explanation for this and the remaining arguments can be found below. wvar : optional Variables for use with weighting functions. wopts : optional Optional input for reusing Chebyshev moments. maxp1 : float or int, optional An upper bound on the number of Chebyshev moments. limlst : int, optional Upper bound on the number of cycles (>=3) for use with a sinusoidal weighting and an infinite end-point. See Also -------- dblquad : double integral tplquad : triple integral nquad : n-dimensional integrals (uses `quad` recursively) fixed_quad : fixed-order Gaussian quadrature quadrature : adaptive Gaussian quadrature odeint : ODE integrator ode : ODE integrator simpson : integrator for sampled data romb : integrator for sampled data scipy.special : for coefficients and roots of orthogonal polynomials Notes ----- For valid results, the integral must converge; behavior for divergent integrals is not guaranteed. **Extra information for quad() inputs and outputs* If full_output is non-zero, then the third output argument (infodict) is a dictionary with entries as tabulated below. For infinite limits, the range is transformed to (0,1) and the optional outputs are given with respect to this transformed range. Let M be the input argument limit and let K be infodict['last']. The entries are: 'neval' The number of function evaluations. 'last' The number, K, of subintervals produced in the subdivision process. 'alist' A rank-1 array of length M, the first K elements of which are the left end points of the subintervals in the partition of the integration range. 'blist' A rank-1 array of length M, the first K elements of which are the right end points of the subintervals. 'rlist' A rank-1 array of length M, the first K elements of which are the integral approximations on the subintervals. 'elist' A rank-1 array of length M, the first K elements of which are the moduli of the absolute error estimates on the subintervals. 'iord' A rank-1 integer array of length M, the first L elements of which are pointers to the error estimates over the subintervals withL=K
ifK<=M/2+2
orL=M+1-K
otherwise. Let I be the sequenceinfodict['iord']
and let E be the sequenceinfodict['elist']
. ThenE[I[1]], ..., E[I[L]]
forms a decreasing sequence. If the input argument points is provided (i.e., it is not None), the following additional outputs are placed in the output dictionary. Assume the points sequence is of length P. 'pts' A rank-1 array of length P+2 containing the integration limits and the break points of the intervals in ascending order. This is an array giving the subintervals over which integration will occur. 'level' A rank-1 integer array of length M (=limit), containing the subdivision levels of the subintervals, i.e., if (aa,bb) is a subinterval of(pts[1], pts[2])
wherepts[0]
andpts[2]
are adjacent elements ofinfodict['pts']
, then (aa,bb) has level l if|bb-aa| = |pts[2]-pts[1]| * 2**(-l)
. 'ndin' A rank-1 integer array of length P+2. After the first integration over the intervals (pts[1], pts[2]), the error estimates over some of the intervals may have been increased artificially in order to put their subdivision forward. This array has ones in slots corresponding to the subintervals for which this happens. Weighting the integrand The input variables, weight and wvar, are used to weight the integrand by a select list of functions. Different integration methods are used to compute the integral with these weighting functions, and these do not support specifying break points. The possible values of weight and the corresponding weighting functions are. ========== =================================== =====================weight
Weight function usedwvar
========== =================================== ===================== 'cos' cos(w*x) wvar = w 'sin' sin(w*x) wvar = w 'alg' g(x) = ((x-a)**alpha)*((b-x)**beta) wvar = (alpha, beta) 'alg-loga' g(x)*log(x-a) wvar = (alpha, beta) 'alg-logb' g(x)*log(b-x) wvar = (alpha, beta) 'alg-log' g(x)*log(x-a)*log(b-x) wvar = (alpha, beta) 'cauchy' 1/(x-c) wvar = c ========== =================================== ===================== wvar holds the parameter w, (alpha, beta), or c depending on the weight selected. In these expressions, a and b are the integration limits. For the 'cos' and 'sin' weighting, additional inputs and outputs are available. For finite integration limits, the integration is performed using a Clenshaw-Curtis method which uses Chebyshev moments. For repeated calculations, these moments are saved in the output dictionary: 'momcom' The maximum level of Chebyshev moments that have been computed, i.e., ifM_c
isinfodict['momcom']
then the moments have been computed for intervals of length|b-a| * 2**(-l)
,l=0,1,...,M_c
. 'nnlog' A rank-1 integer array of length M(=limit), containing the subdivision levels of the subintervals, i.e., an element of this array is equal to l if the corresponding subinterval is|b-a|* 2**(-l)
. 'chebmo' A rank-2 array of shape (25, maxp1) containing the computed Chebyshev moments. These can be passed on to an integration over the same interval by passing this array as the second element of the sequence wopts and passing infodict['momcom'] as the first element. If one of the integration limits is infinite, then a Fourier integral is computed (assuming w neq 0). If full_output is 1 and a numerical error is encountered, besides the error message attached to the output tuple, a dictionary is also appended to the output tuple which translates the error codes in the arrayinfo['ierlst']
to English messages. The output information dictionary contains the following entries instead of 'last', 'alist', 'blist', 'rlist', and 'elist': 'lst' The number of subintervals needed for the integration (call itK_f
). 'rslst' A rank-1 array of length M_f=limlst, whose firstK_f
elements contain the integral contribution over the interval(a+(k-1)c, a+kc)
wherec = (2*floor(|w|) + 1) * pi / |w|
andk=1,2,...,K_f
. 'erlst' A rank-1 array of lengthM_f
containing the error estimate corresponding to the interval in the same position ininfodict['rslist']
. 'ierlst' A rank-1 integer array of lengthM_f
containing an error flag corresponding to the interval in the same position ininfodict['rslist']
. See the explanation dictionary (last entry in the output tuple) for the meaning of the codes. Details of QUADPACK level routines quad calls routines from the FORTRAN library QUADPACK. This section provides details on the conditions for each routine to be called and a short description of each routine. The routine called depends on weight, points and the integration limits a and b. ================ ============== ========== ===================== QUADPACK routine weight points infinite bounds ================ ============== ========== ===================== qagse None No No qagie None No Yes qagpe None Yes No qawoe 'sin', 'cos' No No qawfe 'sin', 'cos' No either a or b qawse 'alg*' No No qawce 'cauchy' No No ================ ============== ========== ===================== The following provides a short desciption from [1]_ for each routine. qagse is an integrator based on globally adaptive interval subdivision in connection with extrapolation, which will eliminate the effects of integrand singularities of several types. qagie handles integration over infinite intervals. The infinite range is mapped onto a finite interval and subsequently the same strategy as inQAGS
is applied. qagpe serves the same purposes as QAGS, but also allows the user to provide explicit information about the location and type of trouble-spots i.e. the abscissae of internal singularities, discontinuities and other difficulties of the integrand function. qawoe is an integrator for the evaluation of \(\int^b_a \cos(\omega x)f(x)dx\) or \(\int^b_a \sin(\omega x)f(x)dx\) over a finite interval [a,b], where \(\omega\) and \(f\) are specified by the user. The rule evaluation component is based on the modified Clenshaw-Curtis technique An adaptive subdivision scheme is used in connection with an extrapolation procedure, which is a modification of that inQAGS
and allows the algorithm to deal with singularities in \(f(x)\). qawfe calculates the Fourier transform \(\int^\infty_a \cos(\omega x)f(x)dx\) or \(\int^\infty_a \sin(\omega x)f(x)dx\) for user-provided \(\omega\) and \(f\). The procedure ofQAWO
is applied on successive finite intervals, and convergence acceleration by means of the \(\varepsilon\)-algorithm is applied to the series of integral approximations. qawse approximate \(\int^b_a w(x)f(x)dx\), with \(a < b\) where \(w(x) = (x-a)^{\alpha}(b-x)^{\beta}v(x)\) with \(\alpha,\beta > -1\), where \(v(x)\) may be one of the following functions: \(1\), \(\log(x-a)\), \(\log(b-x)\), \(\log(x-a)\log(b-x)\). The user specifies \(\alpha\), \(\beta\) and the type of the function \(v\). A globally adaptive subdivision strategy is applied, with modified Clenshaw-Curtis integration on those subintervals which contain a or b. qawce compute \(\int^b_a f(x) / (x-c)dx\) where the integral must be interpreted as a Cauchy principal value integral, for user specified \(c\) and \(f\). The strategy is globally adaptive. Modified Clenshaw-Curtis integration is used on those intervals containing the point \(x = c\). Integration of Complex Function of a Real Variable A complex valued function, \(f\), of a real variable can be written as \(f = g + ih\). Similarly, the integral of \(f\) can be written as .. math:: int_a^b f(x) dx = int_a^b g(x) dx + iint_a^b h(x) dx assuming that the integrals of \(g\) and \(h\) exist over the inteval \([a,b]\) [2]_. Therefore,quad
integrates complex-valued functions by integrating the real and imaginary components separately. References ---------- .. [1] Piessens, Robert; de Doncker-Kapenga, Elise; Überhuber, Christoph W.; Kahaner, David (1983). QUADPACK: A subroutine package for automatic integration. Springer-Verlag. ISBN 978-3-540-12553-2. .. [2] McCullough, Thomas; Phillips, Keith (1973). Foundations of Analysis in the Complex Plane. Holt Rinehart Winston. ISBN 0-03-086370-8 Examples -------- Calculate \(\int^4_0 x^2 dx\) and compare with an analytic result >>> from scipy import integrate >>> import numpy as np >>> x2 = lambda x: x**2 >>> integrate.quad(x2, 0, 4) (21.333333333333332, 2.3684757858670003e-13) >>> print(4**3 / 3.) # analytical result 21.3333333333 Calculate \(\int^\infty_0 e^{-x} dx\) >>> invexp = lambda x: np.exp(-x) >>> integrate.quad(invexp, 0, np.inf) (1.0, 5.842605999138044e-11) Calculate \(\int^1_0 a x \,dx\) for \(a = 1, 3\) >>> f = lambda x, a: a*x >>> y, err = integrate.quad(f, 0, 1, args=(1,)) >>> y 0.5 >>> y, err = integrate.quad(f, 0, 1, args=(3,)) >>> y 1.5 Calculate \(\int^1_0 x^2 + y^2 dx\) with ctypes, holding y parameter as 1:: testlib.c => double func(int n, double args[n]){ return args[0]*args[0] + args[1]*args[1];} compile to library testlib.* :: from scipy import integrate import ctypes lib = ctypes.CDLL('/home/.../testlib.*') #use absolute path lib.func.restype = ctypes.c_double lib.func.argtypes = (ctypes.c_int,ctypes.c_double) integrate.quad(lib.func,0,1,(1)) #(1.3333333333333333, 1.4802973661668752e-14) print((1.0**3/3.0 + 1.0) - (0.0**3/3.0 + 0.0)) #Analytic result # 1.3333333333333333 Be aware that pulse shapes and other sharp features as compared to the size of the integration interval may not be integrated correctly using this method. A simplified example of this limitation is integrating a y-axis reflected step function with many zero values within the integrals bounds. >>> y = lambda x: 1 if x<=0 else 0 >>> integrate.quad(y, -1, 1) (1.0, 1.1102230246251565e-14) >>> integrate.quad(y, -1, 100) (1.0000000002199108, 1.0189464580163188e-08) >>> integrate.quad(y, -1, 10000) (0.0, 0.0)
[((0, xmax), integrate.quad(f1,0,xmax)[0]) for xmax in np.arange(1,5)]
[((0, 1), 0.34858491873298725),
((0, 2), 0.8600106383901718),
((0, 3), 1.0438816972950686),
((0, 4), 1.0074874684274517)]
Por defecto, la rutina devuelve dos valores. El primero es la estimación del valor de la integral y el segundo una estimación del error absoluto . Además, la función acepta límites de integración infinitos (\(\pm \infty\), definidos en Numpy)
integrate.quad(f1,-np.inf,np.inf)
(-0.3871487639489654, 5.459954603228055e-09)
Ejemplo de función fuertemente oscilatoria
k = 200
L = 2*np.pi
a = 0.1
def f2(x):
return np.sin(k*x)*np.exp(-a*x)
# Valor exacto de la integral
I=k/a**2*(np.exp(-a*L)-1)/(1-k**2/a**2)
print(I)
0.0023325601276845158
Iq= integrate.quad(f2,0,L)
/tmp/ipykernel_145715/604810385.py:1: IntegrationWarning: The maximum number of subdivisions (50) has been achieved.
If increasing the limit yields no improvement it is advised to analyze
the integrand in order to determine the difficulties. If the position of a
local difficulty can be determined (singularity, discontinuity) one will
probably gain from splitting up the interval and calling the integrator
on the subranges. Perhaps a special-purpose integrator should be used.
Iq= integrate.quad(f2,0,L)
Iq
(-0.004361082510618083, 0.01911939069137464)
I_err = (I-Iq[0])/I # Error relativo con el valor exacto
print("I= {:.5g} ± {:.5g}\nError relativo= {:.6g}\n".format(*Iq, I_err))
I= -0.0043611 ± 0.019119
Error relativo= 2.86965
El error relativo entre el valor obtenido numéricamente y el valor
exacto I
es grande. Esto se debe a la naturaleza del integrando.
Grafiquemos sólo una pequeña parte
x = np.linspace(0,L,1500)
plt.plot(x, f2(x))
[<matplotlib.lines.Line2D at 0x7fb48216d590>]
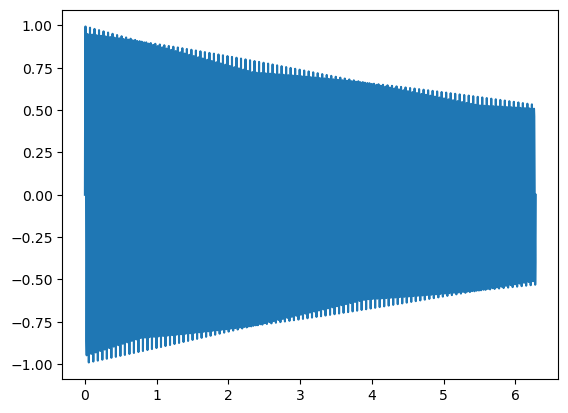
x = np.linspace(0,L/10,1500)
plt.plot(x, f2(x))
[<matplotlib.lines.Line2D at 0x7fb482a811d0>]
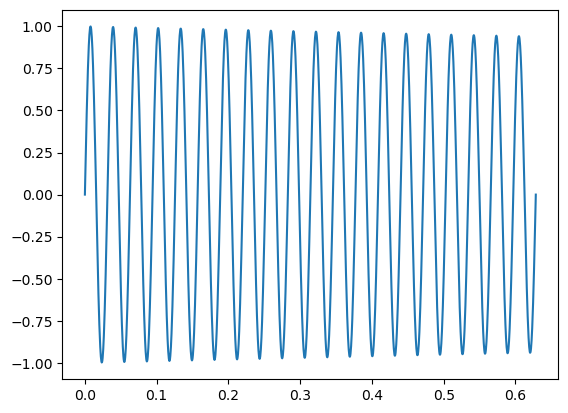
La rutina quad
es versatil y tiene una opción específica para
integrandos oscilatorios, que permite calcular las integrales de una
función \(f\) multiplicadas por una función oscilatoria
Para ello debemos usar el argumento weight
y wvar
. En este caso
usaremos weight='sin'
# La función sin el factor oscilatorio:
def f3(x):
return np.exp(-a*x)
Is = integrate.quad(f3,0,L, weight='sin', wvar=k)
I_err = (I-Is[0])/I # Error relativo con el valor exacto
print("I= {:.5g} ± {:.5g}\nError relativo= {:.6g}\n".format(*Is, I_err))
I= 0.0023326 ± 3.4061e-19
Error relativo= 5e-07
Esto es así, porque una vez que separamos el comportamiento oscilatorio, la función es suave y fácilmente integrable
x = np.linspace(0,L,1500)
plt.plot(x, f3(x))
[<matplotlib.lines.Line2D at 0x7fb4823b6490>]
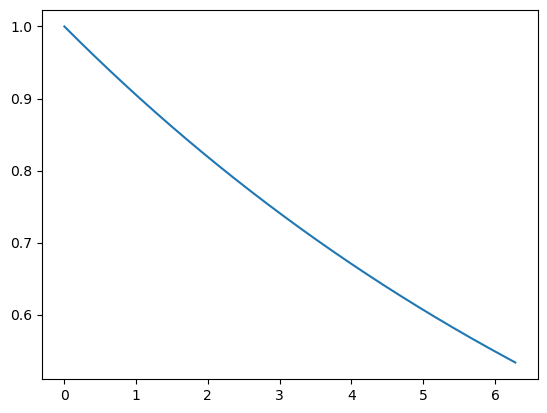
El error relativo obtenido respecto al valor exacto es varios órdenes de magnitud menor. Comparemos los tiempos de ejecución:
%timeit integrate.quad(f2,0,L)
<magic-timeit>:1: IntegrationWarning: The maximum number of subdivisions (50) has been achieved.
If increasing the limit yields no improvement it is advised to analyze
the integrand in order to determine the difficulties. If the position of a
local difficulty can be determined (singularity, discontinuity) one will
probably gain from splitting up the interval and calling the integrator
on the subranges. Perhaps a special-purpose integrator should be used.
4.07 ms ± 251 μs per loop (mean ± std. dev. of 7 runs, 100 loops each)
%timeit integrate.quad(f3,0,L, weight='sin', wvar=k)
26.2 μs ± 1.26 μs per loop (mean ± std. dev. of 7 runs, 10,000 loops each)
Usar un integrador más específico para el integrando no sólo nos da un mejor resultado sino que el tiempo de ejecución es más de 100 veces más corto.
Funciones de más de una variable
Consideremos el caso en que queremos integrar alguna función especial.
Podemos usar Scipy para realizar la integración y para evaluar el
integrando. Como special.jn
depende de dos variables, tenemos que
crear una función intermedia que dependa sólo de la variable de
integración
integrate.quad(lambda x: special.jn(0,x), 0 , 10)
(1.0670113039567362, 7.434789460651883e-14)
En realidad, la función quad
permite el uso de argumentos que se le
pasan a la función a integrar. La forma de llamar al integrador será en
general:
quad(func, a, b, args=(), full_output=0, epsabs=1.49e-08, epsrel=1.49e-08,
limit=50, points=None, weight=None, wvar=None, wopts=None, maxp1=50,
limlst=50)
El argumento args
debe ser una tupla, y contiene los argumentos
extra que acepta la función a integrar, esta función debe llamarse en la
forma func(x, *args)
. O sea que siempre la integramos respecto a su
primer argumento. Apliquemos esto a la función de Bessel. En este caso,
la variable a integrar es el segundo argumento de special.jn
, por lo
que creamos una función con el orden correcto de argumentos:
def bessel_n(x, n):
return special.jn(n,x)
integrate.quad(bessel_n, 0, 10, args=(0,))
(1.0670113039567362, 7.434789460651883e-14)
print(r'n \int_0^10 J_n(x) dx')
for n in range(6):
print(n,': ', integrate.quad(bessel_n, 0, 10, args=(n,))[0])
n int_0^10 J_n(x) dx 0 : 1.0670113039567362 1 : 1.2459357644513482 2 : 0.9800658116190144 3 : 0.7366751370811073 4 : 0.8633070530086401 5 : 1.1758805092851239
Nota
Para calcular integrales múltiples existen rutinas que hacen llamados
sucesivos a la rutina quad()
. Esto incluye rutinas para integrales
dobles (rutina dblquad()
), triples (rutina tplquad()
) y en
general n-dimensionales (rutina nquad()
)
Ejercicios 13 (a)
El submódulo scipy.constants tiene valores de constantes físicas de interés. Usando este módulo compute la constante de Stefan-Boltzmann \(\sigma\) utilizando la relación:
\[\sigma = \frac{2 \pi^5 k_B^4}{15 h^3 c^2}\]Confirme que el valor obtenido es correcto comparando con la constante para esta cantidad en
scipy.constants
Calcular (utilizando
quad
) y graficar para valores de \(k=1,2,5,10\) como función del límite superior \(L\), el valor de las integrales:\[I_{1}(k,L) = \int_{0}^{L} x^{k} e^{-k x / 2} dx\]y
\[I_{2}(k,L) = \int_{0}^{L} x^{k} e^{-k x / 2} \sin{(k x)} dx\]
con rango de variación de \(L\) entre \(0\) y \(2 \pi\).
.
Interpolación y ajuste de curvas (fiteo)
import numpy as np
import matplotlib.pyplot as plt
plt.style.use('presentation')
fsize= (9,6)
Interpolación
Muchas veces tenemos mediciones de datos variando algún parámetro en las condiciones, y estos datos están medidos a intervalos mayores de los que deseamos. En estos casos es común tratar de inferir los valores que tendrían las mediciones para valores intermedios de nuestro parámetro. Una opción es interpolar los datos. Algunas facilidades para ello están en el subpaquete interpolate del paquete Scipy.
Generemos algunos “datos experimentales”
def fmodel(x):
return (np.sin(x))**2*np.exp(-(x/3.5)**2)
x0 = np.linspace(0., 2*np.pi, 60)
y0 = fmodel(x0)
x = np.linspace(0., 2*np.pi, 8)
y = fmodel(x)
plt.plot(x0,y0,'--k', label='función', alpha=0.5)
plt.plot(x,y,'o', markersize=12, label='puntos')
plt.legend(loc='best')
<matplotlib.legend.Legend at 0x7fa88ec081a0>
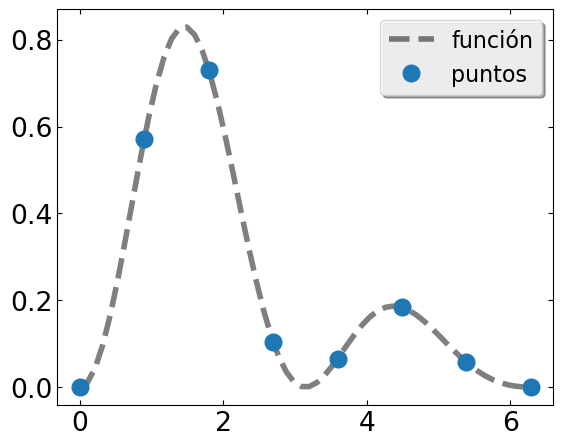
Acá hemos simulado datos con una función oscilante con un decaimiento exponencial.
Ahora, importamos el submódulo interpolate
del módulo scipy
, que
nos permite interpolar los datos:
from scipy import interpolate
La interpolación funciona en dos pasos. En el primer paso realizamos todos los cálculos y obtenemos la función interpolante, y en una segunda etapa utilizamos esa función para interpolar los valores en los nuevos puntos sobre el eje x que necesitamos.
Utilizamos los arrays x
e y
como los pocos “datos
experimentales” obtenidos
print(f"{x = }")
x = array([0. , 0.8975979 , 1.7951958 , 2.6927937 , 3.5903916 ,
4.48798951, 5.38558741, 6.28318531])
y creamos la función interpolante basada en estos puntos:
interpol_lineal = interpolate.interp1d(x, y)
interpol_lineal # función
<scipy.interpolate._interpolate.interp1d at 0x7fa8858dada0>
Ahora, creamos un conjunto de puntos x1
donde queremos evaluar la
función interpolando entre datos medidos
x1 = np.linspace(0, 2*np.pi, 33)
y1_l = interpol_lineal(x1)
plt.plot(x0,y0, '-k', label='función', alpha=0.5)
plt.plot(x, y,'o', markersize=12, label='datos')
plt.plot(x1, y1_l,'--s', markersize=7, label='interp-1d')
plt.legend(loc='best')
<matplotlib.legend.Legend at 0x7fa884aa3b10>
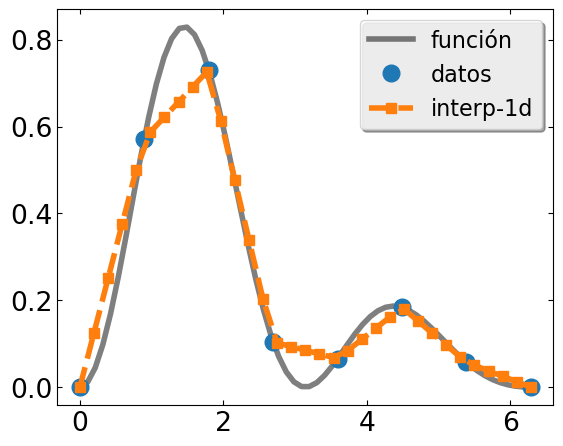
Como vemos, la función que creamos consiste de tramos rectos entre los
puntos “datos”. Para realizar interpolaciones lineales (una recta entre
pares de puntos) también se puede utilizar la rutina interp()
del
módulo Numpy, cuyos argumentos requeridos son: los nuevos puntos
x1
donde queremos interpolar, además de los valores originales de
x
y de y
de la tabla a interpolar:
y1_l2= np.interp(x1,x,y)
Notar que y1_l2
da exactamente los mismos valores que y1_l
np.all(y1_l2 == y1_l)
True
Si bien el uso de np.interp
es más directo, porque no se crea la
función intermedia, cuando creamos la función con interp1d
podemos
aplicarla a diferentes conjuntos de valores de x:
interpol_lineal(np.linspace(0, 2*np.pi, 12))
array([0.00000000e+00, 3.64223643e-01, 6.15515285e-01, 7.16230928e-01,
3.88910634e-01, 9.71667086e-02, 7.27120078e-02, 1.19301459e-01,
1.72109481e-01, 9.17229560e-02, 3.64455561e-02, 2.39038977e-33])
La interface interp1d()
tiene un argumento opcional, kind
, que
define el tipo de interpolación a utilizar. Cuando utilizamos el
argumento ‘nearest’ utiliza para cada valor el más cercano
interpol_near = interpolate.interp1d(x, y, kind='nearest')
y1_n = interpol_near(x1)
plt.plot(x0,y0, '-k', label='función', alpha=0.5)
plt.plot(x, y,'o', markersize=12, label='datos')
plt.plot(x1, y1_l,'--s', markersize=7, label='interp-1d')
plt.plot(x1, y1_n,'.', label='nearest')
plt.legend(loc='best');
print(x1.size, x1.size, x.size)
33 33 8
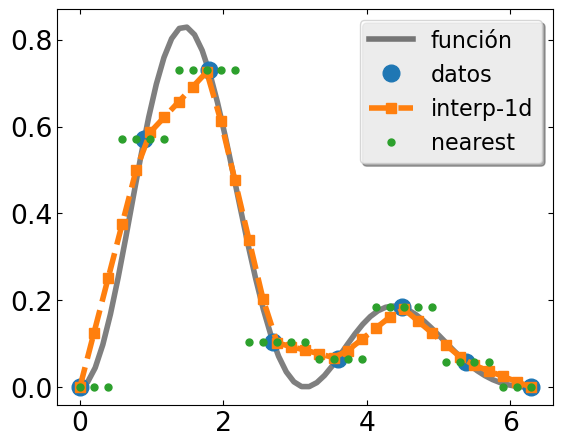
Interpolación con polinomios
Scipy tiene rutinas para interpolar los datos usando un único polinomio global con grado igual al número de puntos dados:
def fm(x):
return x**4 - x**3*np.sin(x/6)
x0 = np.linspace(0., 2*np.pi, 60)
y0 = fm(x0)
x = np.linspace(0., 2*np.pi, 5)
y = fm(x)
#
# Creamos el polinomio interpolador
f = interpolate.lagrange(x, y)
y1 = f(x0)
#
plt.figure(figsize=fsize)
plt.plot(x0,y1,'-', label=f'Lagrange ({x.size})')
plt.plot(x,y,'o', label='datos')
plt.plot(x0,y0,'--', color='C1', label='f(x)', alpha=0.7)
plt.legend(loc='best')
<matplotlib.legend.Legend at 0x7fa884649d10>
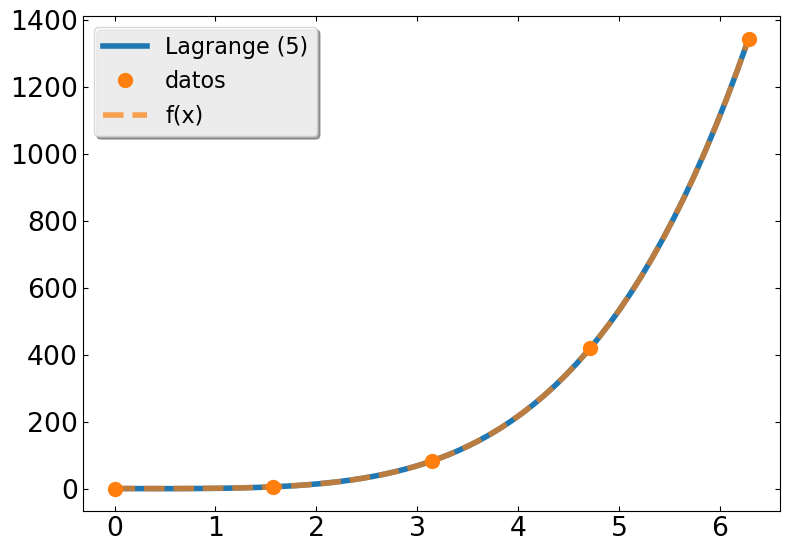
Acá la función lagrange()
devuelve un polinomio del tipo poly1d
,
que puede ser ejecutado (como hicimos en la celda anterior)
type(f)
numpy.poly1d
f.coeffs
array([ 0.9463431 , -0.80568571, 1.98841541, -1.56609363, 0. ])
Los polinomios interpolantes pueden tener problemas, principalmente en
las puntas, o cuando el grado del polinomio es muy alto. Consideremos
por ejemplo el caso donde tenemos una tabla x1 f(x1)
con muchos
datos y queremos interpolar sobre una nueva tabla de valores x0
.
Incluso para un grado intermedio de polinomio se observan oscilaciones
entre los puntos
x0 = np.linspace(0., 2*np.pi, 60)
x1 = np.linspace(0, 2*np.pi, 7)
y1 = fmodel(x1)
f1 = interpolate.lagrange(x1, y1)
plt.figure(figsize=fsize)
plt.plot(x0,f1(x0),'-', label=f'Lagrange ({x1.size})')
plt.plot(x1,y1,'o', label='datos')
plt.plot(x0,fmodel(x0),'--', color='C1', label='f(x)', alpha=0.7)
plt.legend(loc='best')
<matplotlib.legend.Legend at 0x7fa8846defd0>
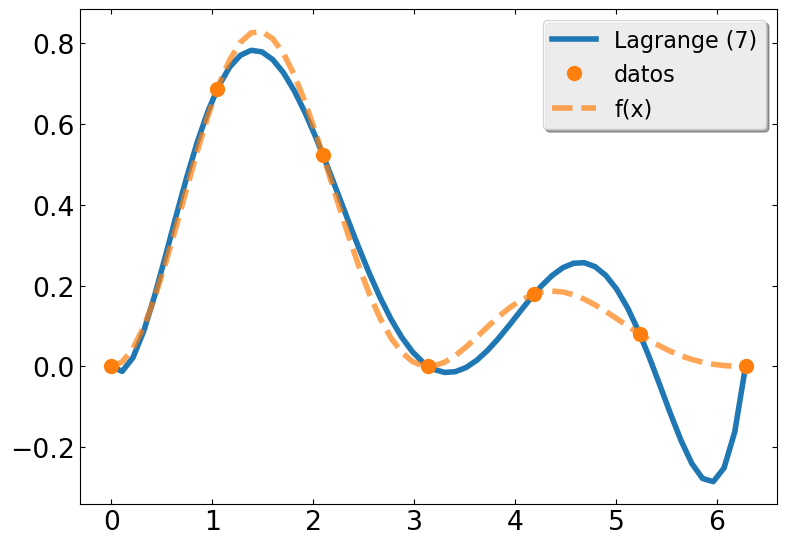
x0 = np.linspace(0., 4*np.pi, 60)
x1 = np.linspace(0, 4*np.pi, 21)
y1 = fmodel(x1)
f1 = interpolate.lagrange(x1, y1)
plt.figure(figsize=fsize)
plt.plot(x0,f1(x0),'-', label=f'Lagrange ({x1.size})')
plt.plot(x1,y1,'o', label='datos')
plt.plot(x0, interpolate.barycentric_interpolate(x1,y1,x0), label="Lagrange (estable)")
plt.plot(x0,fmodel(x0),'--', color='C1', label='f(x)', alpha=0.7)
plt.legend(loc='best')
<matplotlib.legend.Legend at 0x7fa88457f750>
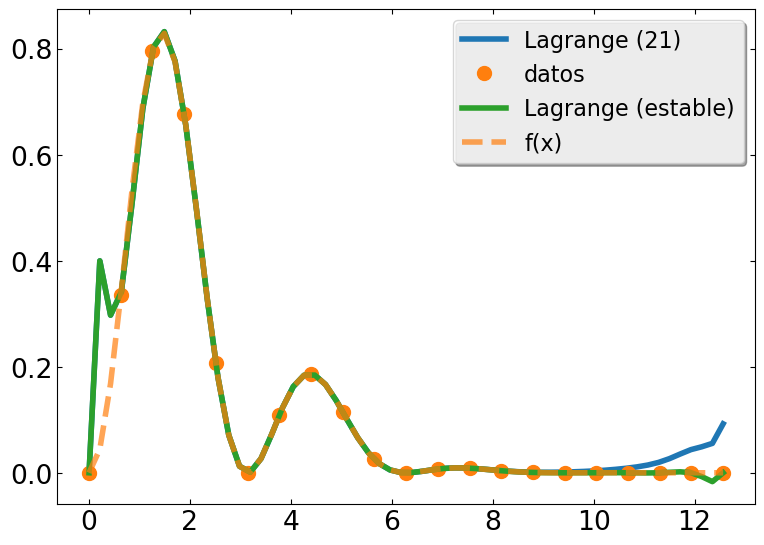
De todas maneras, para los casos en que es aplicable, existen dos
implementaciones: interpolate.lagrange()
y una segunda llamada
interpolate.barycentric_interpolate()
que está basada en un trabajo
de 2004 y es numéricamente más estable.
Splines
Las Splines son interpolaciones por polinomios de a trazos, que se eligen para que no sólo los valores sino también sus derivadas coincidan dando una curva suave.
Para eso, si se pide que la aproximación coincida con los valores tabulados en los puntos dados, la aproximación es efectivamente una interpolación.
Cubic Splines se refiere a que utilizamos polinomios cúbicos en cada trozo.
El argumento opcional kind
de la interface interp1d()
, que
define el tipo de interpolación a utilizar, acepta valores del tipo
string que pueden ser: ‘linear’, ‘nearest’, ‘zero’, ‘slinear’,
‘quadratic’, ‘cubic’, o un número entero indicando el orden.
x0 = np.linspace(0, 2*np.pi, 60)
x1 = np.linspace(0, 2*np.pi, 30)
interp = {}
for k in ['zero', 'slinear', 'quadratic', 'cubic']:
interp[k] = interpolate.interp1d(x, y, kind=k)
fig = plt.figure(figsize=fsize)
plt.plot(x0,y0,'-k', alpha=0.7, label='f(x)')
plt.plot(x,y,'o', markersize=12, label='datos')
plt.plot(x1,interp['cubic'](x1), '--', color='C2', label=u'cúbica')
plt.plot(x1,interp['slinear'](x1),'.-', lw=2, label='lineal')
plt.legend(loc='best')
<matplotlib.legend.Legend at 0x7fa8845ffd90>
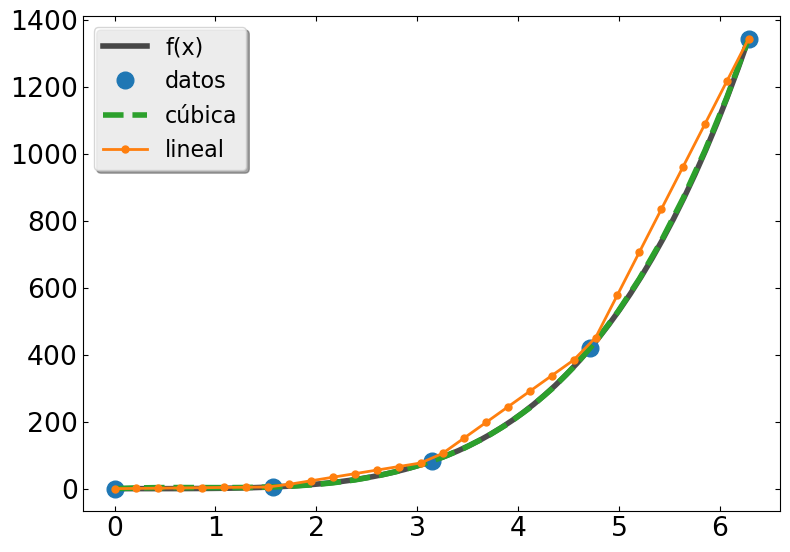
Tratamos de incluir todo en un sólo gráfico (y rogamos que se entienda algo)
plt.figure(figsize=fsize)
for k, v in interp.items():
plt.plot(x1, v(x1), label=k)
plt.plot(x0,y0,'--k', alpha=0.7, label='f(x)')
plt.plot(x,y,'ob', markersize=12, label='datos')
plt.legend(loc='best')
<matplotlib.legend.Legend at 0x7fa8844b9810>
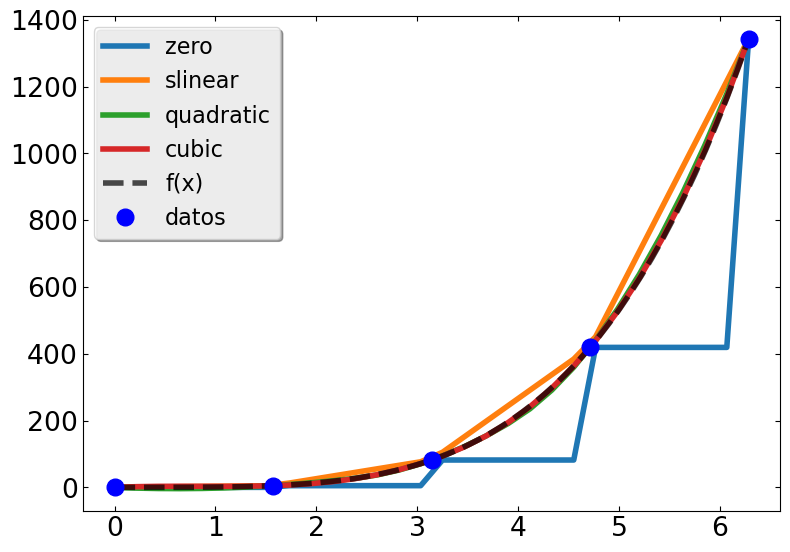
En resumen, los métodos disponibles en interpolate.interp1d
son:
linear
: Interpolación lineal, utilizando rectas (default)nearest
: Valor constante correspondiente al dato más cercanozero
o0
: Una spline de orden cero. Toma el valor a la izquierdaslinear
o1
: Spline de orden 1. Igual a ‘linear’quadratic
o2
: Spline de segundo ordencubic
o3
: Spline de tercer orden
Como vemos de los argumentos zero
, slinear
, quadratic
,
cubic
para especificar splines de cero, primer, segundo y tercer
orden se puede pasar como argumento un número. En ese caso se utiliza
siempre splines y el número indica el orden de las splines a
utilizar:
for k,s in zip([0,1,2,3], ['zero','slinear','quadratic','cubic']):
num = interpolate.interp1d(x,y, kind=k)
tipo = interpolate.interp1d(x,y, kind=s)
print(f"¿{k} == {s}? -> {np.allclose(num(x1), tipo(x1))}")
¿0 == zero? -> True
¿1 == slinear? -> True
¿2 == quadratic? -> True
¿3 == cubic? -> True
Además La interpolación lineal simple es, en la práctica, igual a la interpolación por splines de primer orden:
interpol_lineal = interpolate.interp1d(x, y)
np.allclose(interp['slinear'](x1), interpol_lineal(x1)) # También son iguales
True
Finalmente, veamos que la interpolación nearest
toma para cada nuevo
valor x1
el valor y1(x1)
igual a y(x0)
donde x0
es el
valor más cercano a x1
mientras que la spline de orden cero
(zero
) toma el valor más cercano a la izquierda del dato:
interpol_near = interpolate.interp1d(x, y, kind='nearest')
alfa=1
plt.figure(figsize=fsize)
plt.plot(x1, interpol_near(x1),'-o', label='nearest', alpha=alfa)
plt.plot(x1, interp['zero'](x1), '-.', label='Spline zero'.format(k), alpha=alfa)
plt.plot(x,y,'xk', markersize=12, label='datos')
plt.legend(loc='best');
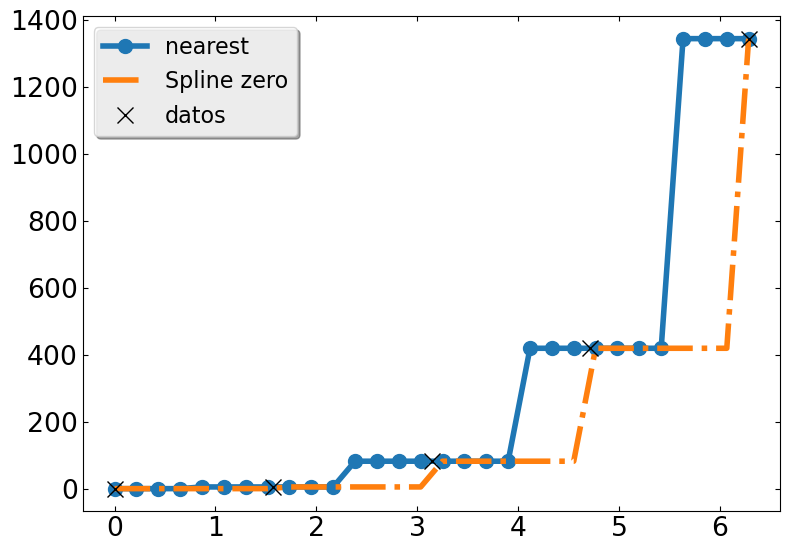
El submódulo signal
tiene rutinas adicionales para realizar
splines, que permiten agregar un “alisado”, pero en este caso ya no
interpolan estrictamente sino que puede ser que la aproximación no pase
por los puntos dados.
B-Splines
Hay otra opción para realizar interpolación con Splines en Scipy. Las llamadas B-Splines son funciones diseñadas para generalizar polinomios, con un alto grado de localidad.
Para definir las B-Splines necesitamos dos cosas:
Elegir el grado de los polinomios (mayor o igual a 0)
Dividir el intervalo en \(n\) “nodos”
Las funciones se definen mediante la recursión:
Las más simples, cuando el orden es k=0, son funciones constantes a trozos
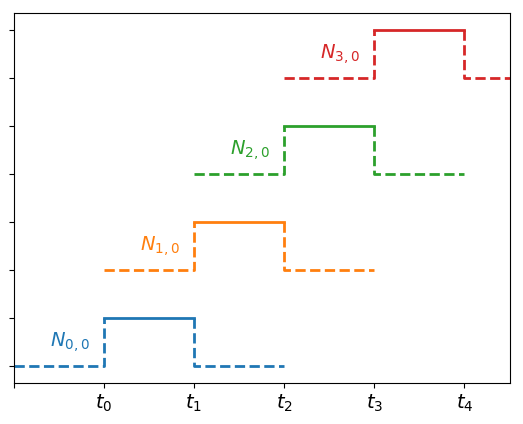
Para \(k>0\) las funciones se calculan por recurrencia en término de dos funciones del orden anterior. Entonces, siempre serán diferentes de cero sólo en un intervalo finito. En ese intervalo presentan un único máximo y luego decaen suavemente. Las más usuales son las de orden \(k=3\):
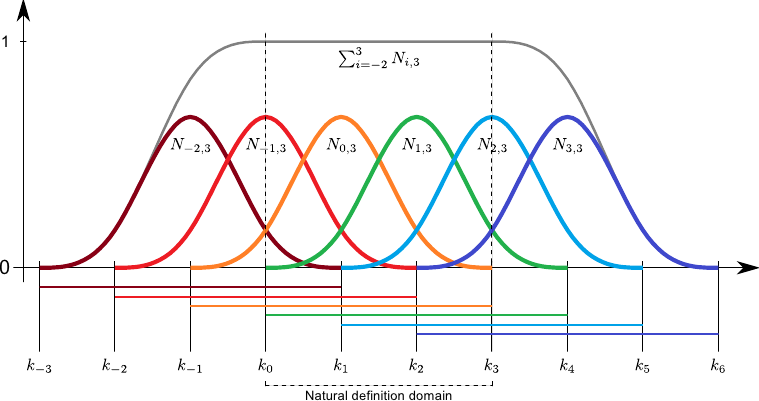
(Figura de http://www.brnt.eu/phd)
La idea es encontrar una función \(f(x)\) que sea suave y pase por la tabla de puntos \((x,y)\) dados, o cerca de ellos con la condición que tanto la función como algunas de sus derivadas sea suave. La función \(f(x)\) se describe como una expansión en la base de Splines (y de ahí el nombre B-Splines)
La aproximación se elige de tal manera de optimizar el número de elementos de la base a utilizar con la condición que el error cuadrático a los puntos sea menor a un cierto valor umbral \(s\)
Veamos cómo usar la implementación de Scipy para interpolar datos. En primer lugar creamos la representación en B-Splines de nuestra tabla de datos \((x,y)\):
tck0 = interpolate.splrep(x,y)
Acá, otra vez estamos operando en dos pasos. En el primero creamos la
representación de las splines para los datos dados. Como no pusimos
explícitamente el orden, utiliza el valor por default k=3
.
En el segundo paso obtenemos los valores interpolados sobre la grilla
x2
:
tck0
(array([0. , 0. , 0. , 0. , 3.14159265,
6.28318531, 6.28318531, 6.28318531, 6.28318531]),
array([-1.10164975e-15, 1.37237275e+01, -6.86554640e+01, 4.51211011e+02,
1.34372767e+03, 0.00000000e+00, 0.00000000e+00, 0.00000000e+00,
0.00000000e+00]),
3)
x2 = np.linspace(0, 2*np.pi, 60) # Nuevos puntos donde interpolar
y_s0 = interpolate.splev(x2, tck0) # Valores interpolados: y_s0[j] = f(x2[j])
plt.figure(figsize=fsize)
plt.plot(x,y,'o', markersize=12, label='datos')
plt.plot(x2,y_s0,'-', label=r'B-Spline')
plt.plot(x0,y0,'--k', alpha=0.7, label='f(x)')
plt.legend(loc='best');
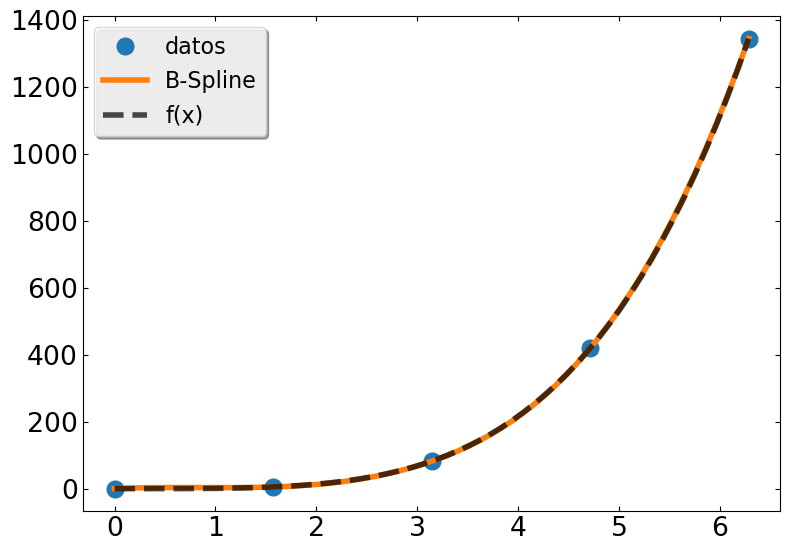
Estas funciones interpolan los datos con curvas continuas y con derivadas segundas continuas.
Lines are guides to the eyes
Sin embargo, estas rutinas no necesariamente realizan interpolación en forma estricta, pasando por todos los puntos dados, sino que en realidad realiza una aproximación minimzando por cuadrados mínimos la distancia a la tabla de puntos dados.
Esto es particularmente importante cuando tenemos datos que tienen dispersión. En esos casos necesitamos curvas que no interpolen, es decir que no necesariamente pasen por todos los puntos.
splrep
tiene varios argumentos opcionales. Entre ellos
un parámetro de suavizado s
que corresponde a la condición de
distancia entre la aproximación y los valores de la tabla mencionado
anteriormente.x, y
con
\(x \in [0,2\pi]\) no necesariamente equiespaciados,
\(y=\sin(x)/2\), donde le agregamos algo de ruido a y
# Creamos dos tablas de valores x, y
x3 = np.linspace(0., 2*np.pi, 40)
x4 = np.linspace(0., 2*np.pi, 40)
x3[5:-5] -= 0.7*(0.5-np.random.random(30)) # Le agregamos una separación al azar
x3.sort() # Los ordenamos
plt.plot(x3-x4,'o',label='data')
plt.plot(x3-x3, '-', alpha=0.7,label='sin incerteza')
plt.ylim((-0.3,0.3))
plt.legend();
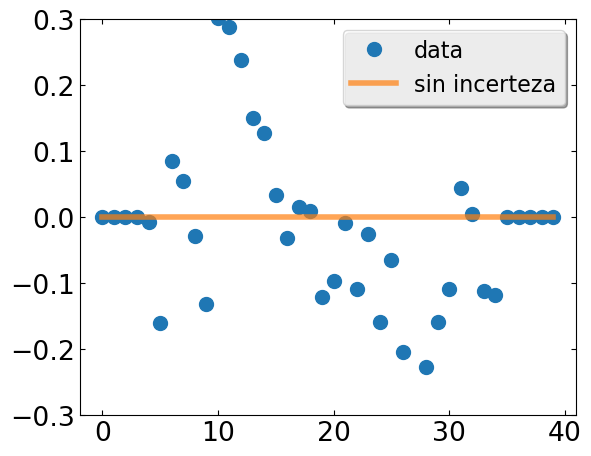
y4 = 0.5* np.sin(x4)
y3 = 0.5* np.sin(x3) * (1+ 0.6*(0.5-np.random.random(x3.size)))
# Los puntos a interpolar tienen "ruido" en las dos direcciones x-y
plt.plot(x3,y3,'o', x4,y4, '.', alpha=0.7 );
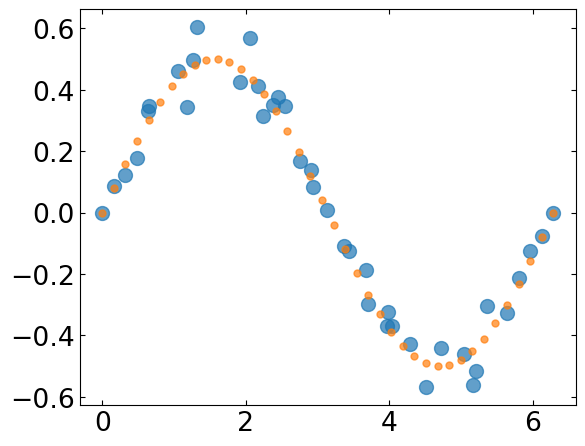
# Grilla donde evaluar la función interpolada
x1 = np.linspace(0, 2*np.pi, 90)
tck0 = interpolate.splrep(x3,y3, s=0) # Interpolación con B-Splines
y_s0 = interpolate.splev(x1,tck0) # Evaluamos en la nueva grilla
tck3 = interpolate.splrep(x3,y3,s=0.3) # Aproximación suavizada
y_s3 = interpolate.splev(x1,tck3) # Evaluamos en la nueva grilla
plt.figure(figsize=fsize)
plt.plot(x1,y_s0,'--', lw=3, label=u'interpolación' )
plt.plot(x1,y_s3, "-", label=u'suavizado');
plt.plot(x3,y3,'o', label='datos' )
plt.legend(loc='best');
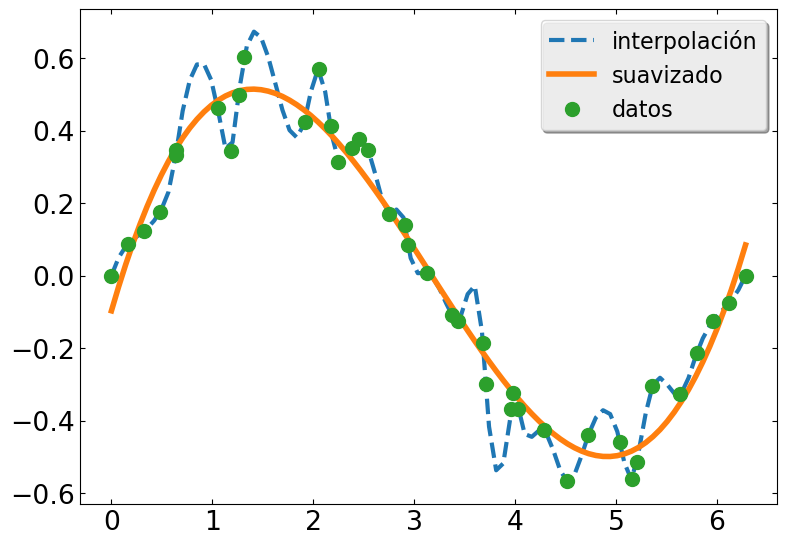
El valor del parámetro s
determina cuanto se suaviza la curva. El
valor por default s=0
obliga al algoritmo a obtener una solución que
no difiere en los valores de la tabla, un valor de s
mayor que cero
da cierta libertad para obtener la aproximación que se acerque a todos
los valores manteniendo una curva relativamente suave. El suavizado
máximo corresponde a s=1
. Veamos cómo cambia la aproximación con el
factor s
:
tck1 = interpolate.splrep(x3,y3, s=0.1) # Interpolación con suavizado
y_s1 = interpolate.splev(x1,tck1)
plt.figure(figsize=fsize)
plt.plot(x1,y_s1, "-", label='s=0.1');
plt.plot(x1,y_s3, "--", label='s=0.3');
plt.plot(x3,y3,'o', markersize=8, label='datos' )
plt.legend(loc='best');
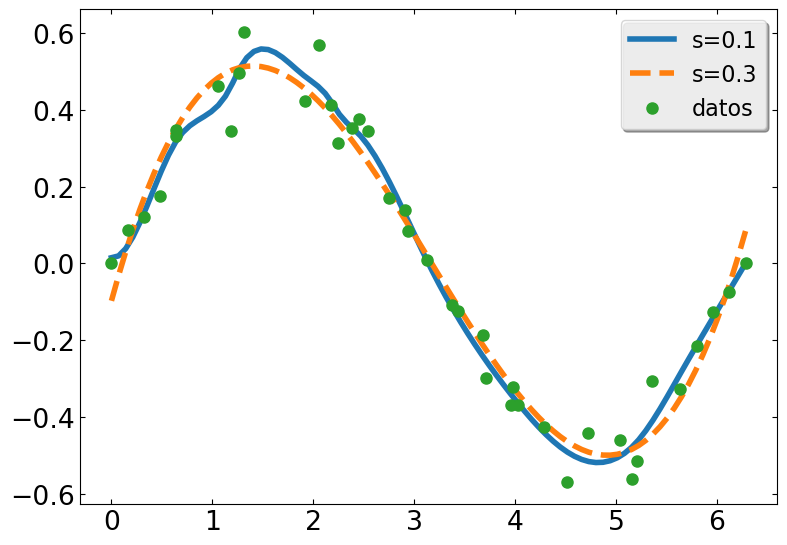
fig, (ax0, ax1) = plt.subplots(figsize=(2.1*fsize[0], fsize[1]), ncols=2)
for s in [0, 0.01, 0.05, 0.1, 0.2, 0.5]:
tck1 = interpolate.splrep(x3,y3, s=s) # Interpolación con suavizado
ys = interpolate.splev(x1,tck1)
ax0.plot(x1,ys, "-", label=f'{s =}');
ax1.plot(x1,ys, "-", label=f'{s =}');
ax0.plot(x3,y3,'ok', label='datos' )
ax1.plot(x3,y3,'ok', label='datos' )
plt.xlim(3,6)
ax1.set_ylim(-0.9, -0.2)
ax1.legend(loc='best');
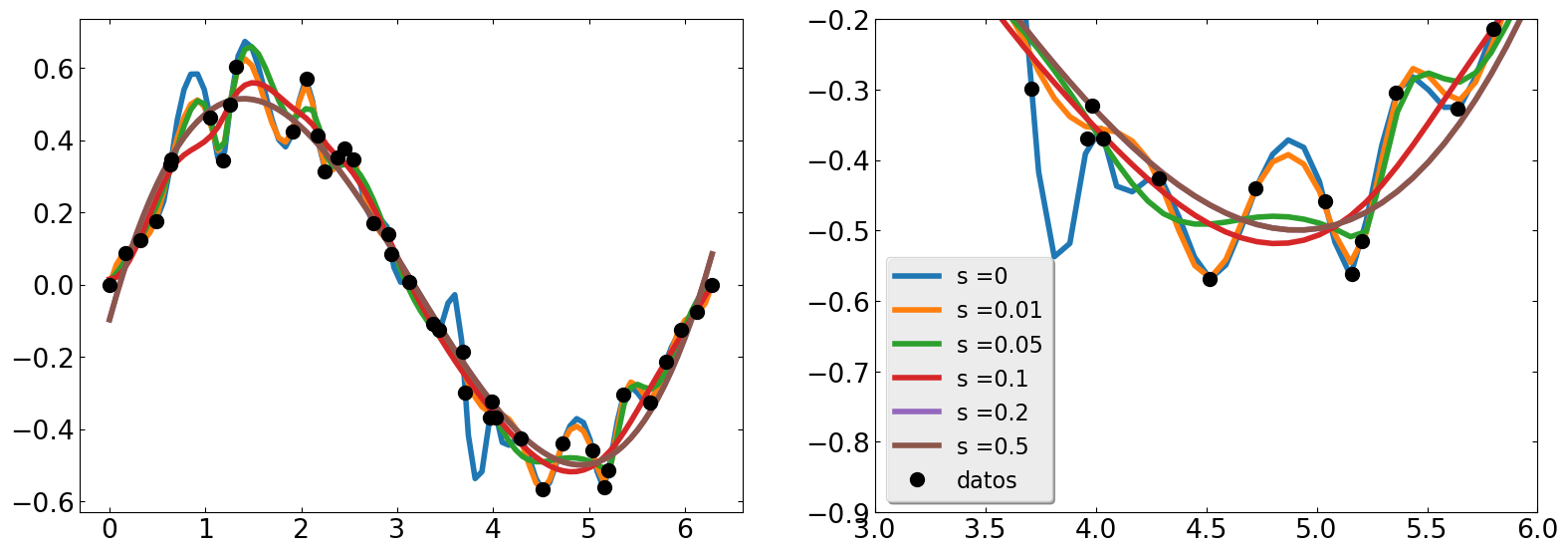
Cantidades derivadas de splines
De la interpolación (suavizada) podemos calcular, por ejemplo, la derivada.
yderiv = interpolate.splev(x1,tck3,der=1) # Derivada
Si tenemos sólo los datos podríamos tratar de calcular la derivada en forma numérica como el coseno
Comparemos los dos resultados:
cond = (x3 > np.pi/2) & (x3 < 3*np.pi/2)
yprima1 = np.where(cond, -1, 1) * 0.5*np.sqrt(np.abs(1 - (2*y3)**2))
plt.figure(figsize=fsize)
plt.plot(x3, yprima1,"sr", label=r"con $\sqrt{1-(2y)^2}/2$")
plt.plot(x1,yderiv,'-k', label=u'Splines')
plt.legend(loc='best');
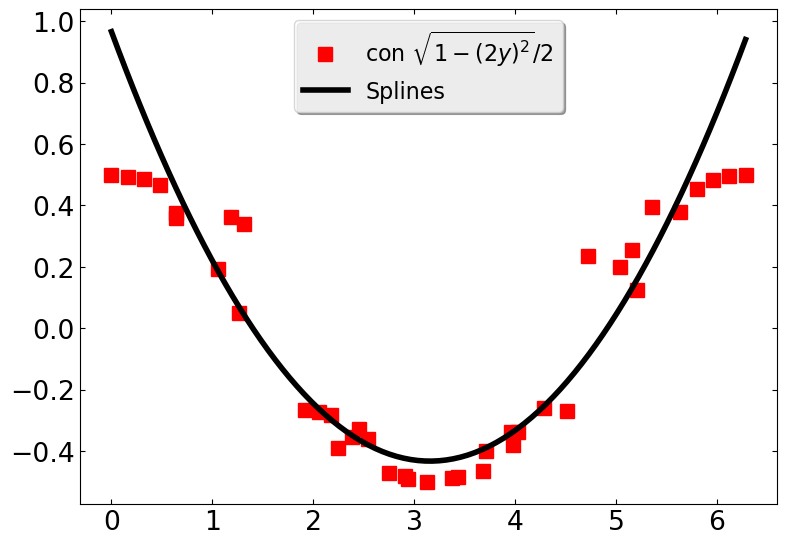
o podemos calcular la integral, o las raíces de la función
plt.figure(figsize=fsize)
yt= np.array([interpolate.splint(0,t,tck3) for t in x1])
plt.plot(x1,yt,'o');
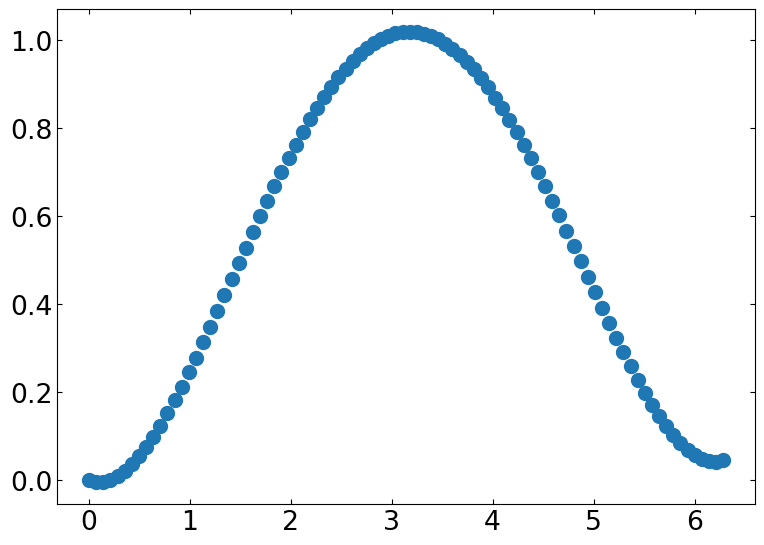
raices = interpolate.sproot(tck3)
plt.axhline(0, color='k',ls='--');
plt.plot(x3,y3, "--", label=u'datos');
plt.plot(x1,y_s3, "-", label=u's=0.3');
plt.plot(raices, np.zeros(len(raices)), 'o', markersize=12)
plt.legend();
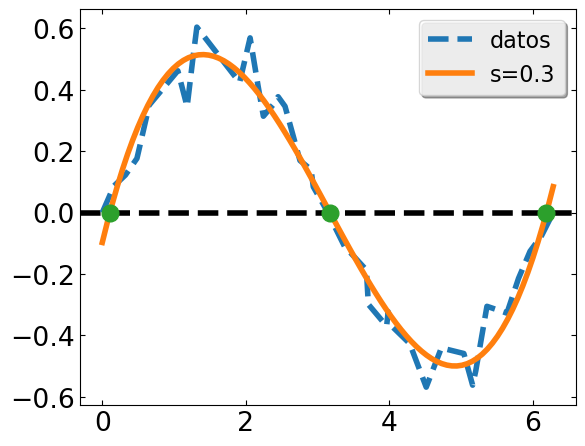
# Generamos los datos
def f(x, y):
s = np.hypot(x, y) # Calcula la hipotenusa
phi = np.arctan2(y, x) # Calcula el ángulo
tau = s + s*(1-s)/5 * np.sin(6*phi)
return 5*(1-tau) + tau
# Generamos los puntos x,y,z en una grilla para comparar con la interpolación
# Notar que es una grilla de 100x100 = 10000 puntos
#
x = np.linspace(-1,1,100)
y = np.linspace(-1,1,100)
X, Y = np.meshgrid(x,y)
T = f(X, Y)
Aquí T
contiene la función sobre todos los puntos \((x,y)\)
obtenidos de combinar cada punto en el vector x
con cada punto en el
vector y
. Notar que la cantidad total de puntos de T
es
T.size = 10000
Elegimos npts=400
puntos al azar de la función que vamos a usar como
tabla de datos a interpolar usando distintos métodos de interpolación
npts = 400
px, py = np.random.choice(x, npts), np.random.choice(y, npts)
Z = f(px, py)
Para poder mostrar todos los métodos juntos, vamos a interpolar dentro
del loop for
# Graficación:
fig, ax = plt.subplots(nrows=2, ncols=2, figsize=fsize, )
# Graficamos la función sobre la grilla estructurada a modo de ilustración
# Graficamos los puntos seleccionados
ax[0,0].contourf(X, Y, T)
ax[0,0].scatter(px, py, c='k', alpha=0.6, marker='.', s=8)
ax[0,0].set_title('Puntos de f(X,Y)', fontsize='large')
# Interpolamos usando los distintos métodos y graficamos
for i, method in enumerate(('nearest', 'linear', 'cubic')):
Ti = interpolate.griddata((px, py), Z, (X, Y), method=method)
r, c = (i+1) // 2, (i+1) % 2
ax[r,c].contourf(X, Y, Ti)
ax[r,c].set_title('{}'.format(method), fontsize='large')
plt.subplots_adjust(hspace=0.3, wspace=0.3)
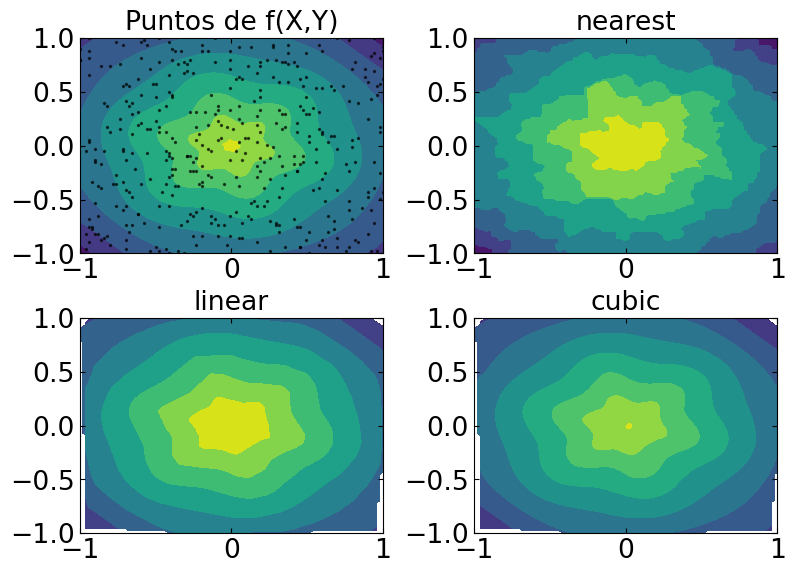
En la primera figura (arriba a la izquierda) graficamos la función evaluada en el total de puntos (10000 puntos) junto con los puntos utilizados para el ajuste. Los otros tres gráficos corresponden a la función evaluada siguiendo el ajuste correspondiente en cada caso.
Fiteos de datos
Ajuste con polinomios
Habitualmente realizamos ajustes sobre datos que tienen incertezas o ruido. Generemos estos datos (con ruido normalmente distribuido)
plt.figure(figsize=fsize)
x = np.linspace(0, 10, 50)
y0 = 2.5*x + 1.2
ruido = np.random.normal(loc= 0., scale= 1, size= y0.size)
y = y0 + ruido
plt.plot(x,y0,'--')
plt.plot(x,y, 'ok', alpha=0.6)
plt.xlabel("Eje x")
plt.ylabel("Eje y");
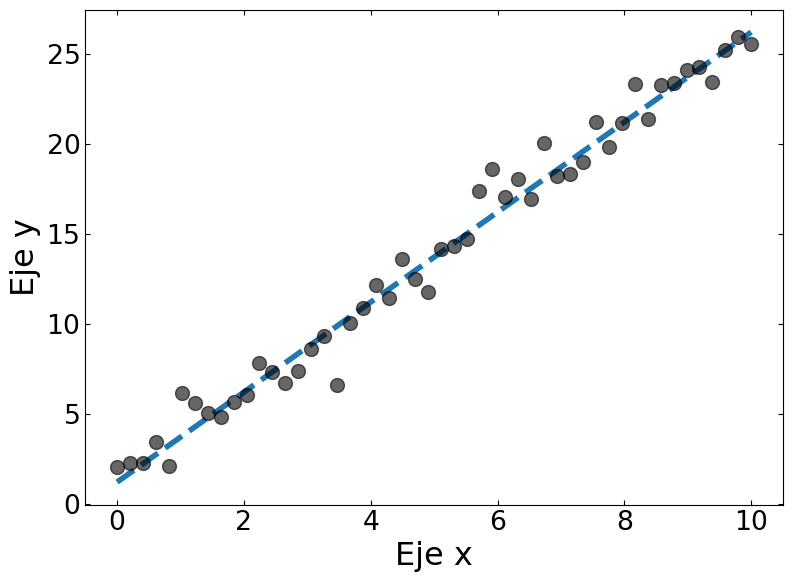
Ahora vamos a ajustar con una recta
Es una regresión lineal (o una aproximación con polinomios de primer orden)
p = np.polyfit(x,y,1)
# np.info(np.polyfit) # para obtener más información
print(p)
print(type(p)) # Qué tipo es?
[2.49011694 1.34883398]
<class 'numpy.ndarray'>
Nota
¿Qué devuelve polyfit()
?
Un array correspondiente a los coeficientes del polinomio de fiteo. En este caso, como estamos haciendo un ajuste lineal, nos devuelve los coeficientes de un polinomio de primer orden (una recta)
y = p[0]*x + p[1]
np.polyfit?
[0;31mSignature:[0m [0mnp[0m[0;34m.[0m[0mpolyfit[0m[0;34m([0m[0mx[0m[0;34m,[0m [0my[0m[0;34m,[0m [0mdeg[0m[0;34m,[0m [0mrcond[0m[0;34m=[0m[0;32mNone[0m[0;34m,[0m [0mfull[0m[0;34m=[0m[0;32mFalse[0m[0;34m,[0m [0mw[0m[0;34m=[0m[0;32mNone[0m[0;34m,[0m [0mcov[0m[0;34m=[0m[0;32mFalse[0m[0;34m)[0m[0;34m[0m[0;34m[0m[0m [0;31mCall signature:[0m [0mnp[0m[0;34m.[0m[0mpolyfit[0m[0;34m([0m[0;34m*[0m[0margs[0m[0;34m,[0m [0;34m**[0m[0mkwargs[0m[0;34m)[0m[0;34m[0m[0;34m[0m[0m [0;31mType:[0m _ArrayFunctionDispatcher [0;31mString form:[0m <function polyfit at 0x7f09d8050900> [0;31mFile:[0m /usr/lib64/python3.13/site-packages/numpy/lib/polynomial.py [0;31mDocstring:[0m Least squares polynomial fit. .. note:: This forms part of the old polynomial API. Since version 1.4, the new polynomial API defined in numpy.polynomial is preferred. A summary of the differences can be found in the transition guide. Fit a polynomialp(x) = p[0] * x**deg + ... + p[deg]
of degree deg to points (x, y). Returns a vector of coefficients p that minimises the squared error in the order deg, deg-1, ... 0. The Polynomial.fit <numpy.polynomial.polynomial.Polynomial.fit> class method is recommended for new code as it is more stable numerically. See the documentation of the method for more information. Parameters ---------- x : array_like, shape (M,) x-coordinates of the M sample points(x[i], y[i])
. y : array_like, shape (M,) or (M, K) y-coordinates of the sample points. Several data sets of sample points sharing the same x-coordinates can be fitted at once by passing in a 2D-array that contains one dataset per column. deg : int Degree of the fitting polynomial rcond : float, optional Relative condition number of the fit. Singular values smaller than this relative to the largest singular value will be ignored. The default value is len(x)*eps, where eps is the relative precision of the float type, about 2e-16 in most cases. full : bool, optional Switch determining nature of return value. When it is False (the default) just the coefficients are returned, when True diagnostic information from the singular value decomposition is also returned. w : array_like, shape (M,), optional Weights. If not None, the weightw[i]
applies to the unsquared residualy[i] - y_hat[i]
atx[i]
. Ideally the weights are chosen so that the errors of the productsw[i]*y[i]
all have the same variance. When using inverse-variance weighting, usew[i] = 1/sigma(y[i])
. The default value is None. cov : bool or str, optional If given and not False, return not just the estimate but also its covariance matrix. By default, the covariance are scaled by chi2/dof, where dof = M - (deg + 1), i.e., the weights are presumed to be unreliable except in a relative sense and everything is scaled such that the reduced chi2 is unity. This scaling is omitted ifcov='unscaled'
, as is relevant for the case that the weights are w = 1/sigma, with sigma known to be a reliable estimate of the uncertainty. Returns ------- p : ndarray, shape (deg + 1,) or (deg + 1, K) Polynomial coefficients, highest power first. If y was 2-D, the coefficients for k-th data set are inp[:,k]
. residuals, rank, singular_values, rcond These values are only returned iffull == True
- residuals -- sum of squared residuals of the least squares fit - rank -- the effective rank of the scaled Vandermonde coefficient matrix - singular_values -- singular values of the scaled Vandermonde coefficient matrix - rcond -- value of rcond. For more details, see numpy.linalg.lstsq. V : ndarray, shape (M,M) or (M,M,K) Present only iffull == False
andcov == True
. The covariance matrix of the polynomial coefficient estimates. The diagonal of this matrix are the variance estimates for each coefficient. If y is a 2-D array, then the covariance matrix for the k-th data set are inV[:,:,k]
Warns ----- RankWarning The rank of the coefficient matrix in the least-squares fit is deficient. The warning is only raised iffull == False
. The warnings can be turned off by >>> import warnings >>> warnings.simplefilter('ignore', np.RankWarning) See Also -------- polyval : Compute polynomial values. linalg.lstsq : Computes a least-squares fit. scipy.interpolate.UnivariateSpline : Computes spline fits. Notes ----- The solution minimizes the squared error .. math:: E = sum_{j=0}^k |p(x_j) - y_j|^2 in the equations:: x[0]**n * p[0] + ... + x[0] * p[n-1] + p[n] = y[0] x[1]**n * p[0] + ... + x[1] * p[n-1] + p[n] = y[1] ... x[k]**n * p[0] + ... + x[k] * p[n-1] + p[n] = y[k] The coefficient matrix of the coefficients p is a Vandermonde matrix. polyfit issues a RankWarning when the least-squares fit is badly conditioned. This implies that the best fit is not well-defined due to numerical error. The results may be improved by lowering the polynomial degree or by replacing x by x - x.mean(). The rcond parameter can also be set to a value smaller than its default, but the resulting fit may be spurious: including contributions from the small singular values can add numerical noise to the result. Note that fitting polynomial coefficients is inherently badly conditioned when the degree of the polynomial is large or the interval of sample points is badly centered. The quality of the fit should always be checked in these cases. When polynomial fits are not satisfactory, splines may be a good alternative. References ---------- .. [1] Wikipedia, "Curve fitting", https://en.wikipedia.org/wiki/Curve_fitting .. [2] Wikipedia, "Polynomial interpolation", https://en.wikipedia.org/wiki/Polynomial_interpolation Examples -------- >>> import warnings >>> x = np.array([0.0, 1.0, 2.0, 3.0, 4.0, 5.0]) >>> y = np.array([0.0, 0.8, 0.9, 0.1, -0.8, -1.0]) >>> z = np.polyfit(x, y, 3) >>> z array([ 0.08703704, -0.81349206, 1.69312169, -0.03968254]) # may vary It is convenient to use poly1d objects for dealing with polynomials: >>> p = np.poly1d(z) >>> p(0.5) 0.6143849206349179 # may vary >>> p(3.5) -0.34732142857143039 # may vary >>> p(10) 22.579365079365115 # may vary High-order polynomials may oscillate wildly: >>> with warnings.catch_warnings(): ... warnings.simplefilter('ignore', np.RankWarning) ... p30 = np.poly1d(np.polyfit(x, y, 30)) ... >>> p30(4) -0.80000000000000204 # may vary >>> p30(5) -0.99999999999999445 # may vary >>> p30(4.5) -0.10547061179440398 # may vary Illustration: >>> import matplotlib.pyplot as plt >>> xp = np.linspace(-2, 6, 100) >>> _ = plt.plot(x, y, '.', xp, p(xp), '-', xp, p30(xp), '--') >>> plt.ylim(-2,2) (-2, 2) >>> plt.show() [0;31mClass docstring:[0m Class to wrap functions with checks for __array_function__ overrides. All arguments are required, and can only be passed by position. Parameters ---------- dispatcher : function or None The dispatcher function that returns a single sequence-like object of all arguments relevant. It must have the same signature (except the default values) as the actual implementation. IfNone
, this is alike=
dispatcher and the_ArrayFunctionDispatcher
must be called withlike
as the first (additional and positional) argument. implementation : function Function that implements the operation on NumPy arrays without overrides. Arguments passed calling the_ArrayFunctionDispatcher
will be forwarded to this (and thedispatcher
) as if using*args, **kwargs
. Attributes ---------- _implementation : function The original implementation passed in.
plt.figure(figsize=fsize)
plt.plot(x, p[0]*x + p[1], '-', label='Ajuste')
plt.plot(x,y,'ok', label='datos', alpha=0.6)
plt.legend(loc='best');
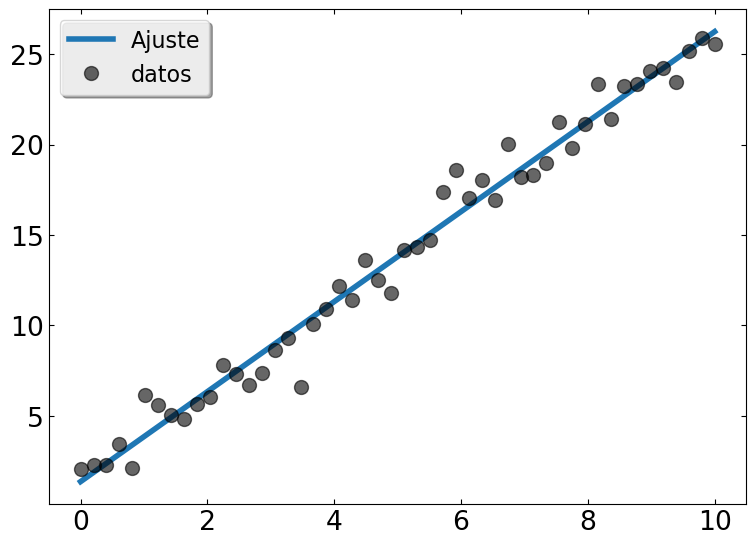
Ahora en vez de escribir la recta explícitamente le pedimos a numpy que lo haga usando los coeficientes que encontramos mediante el fiteo (utilizando la función polyval)
y = np.polyval(p,x)
plt.figure(figsize=fsize)
plt.plot(x, np.polyval(p,x), '-', label='Ajuste')
plt.plot(x,y,'ok', label='datos', alpha=0.6)
plt.legend(loc='best');
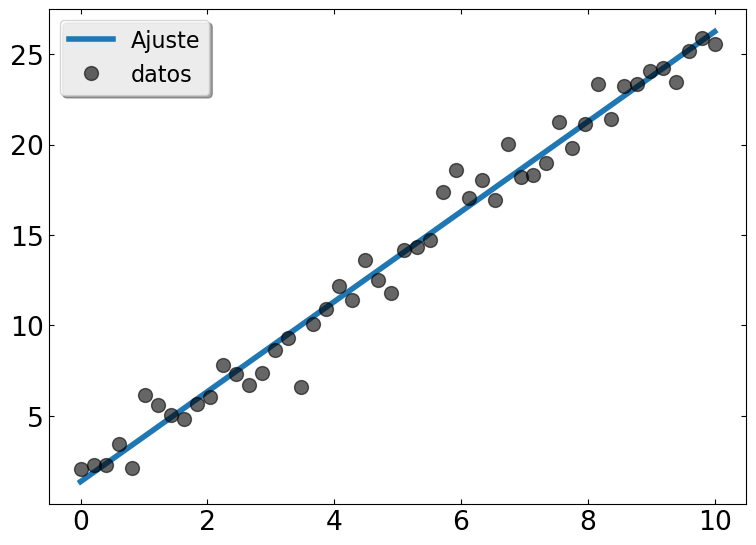
Como vemos, arroja exactamente el mismo resultado.
Si los datos tienen mucho ruido lo que obtenemos es, por supuesto, una recta que pasa por la nube de puntos:
y= y0 + 5*ruido
p = np.polyfit(x, y , 1)
plt.figure(figsize=fsize)
plt.plot(x,np.polyval(p,x),'--', label='Ajuste')
plt.plot(x,y, 'ok', alpha=0.6, label='datos')
plt.legend(loc='best');
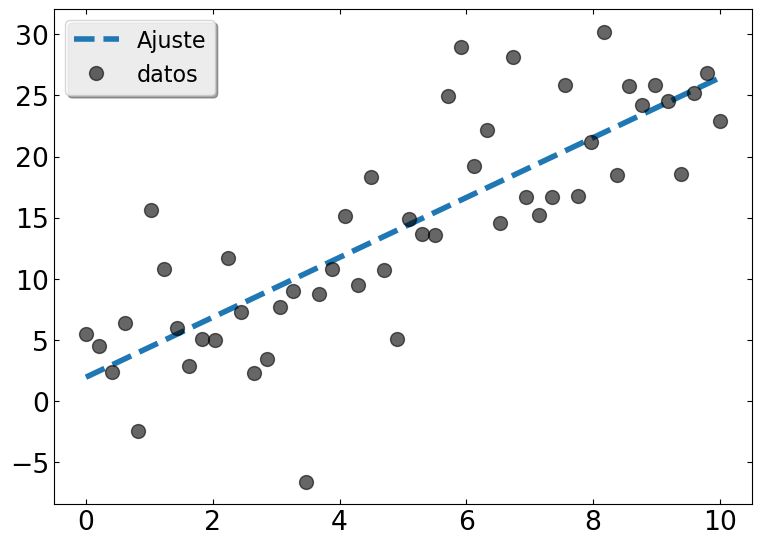
p
array([2.45058468, 1.94416991])
Similarmente podemos usar polinomios de orden superior. Por ejemplo,
para utilizar parábolas sólo tenemos que cambiar el orden n
del
polinomio en el argumento de polyfit(x, y, n)
:
# Generamos los datos
a = [2.5, 1.4, 3.]
y0 = np.polyval(a,x)
y = y0 + 10*ruido
# Ajustamos con un polinomio de segundo grado
p = np.polyfit(x, y , 2)
plt.figure(figsize=fsize)
plt.plot(x,y0,'-', label="$f(x)={0:.2}x^2 + {1:.2} x + {2:.2}$".format(*a))
plt.plot(x,np.polyval(p,x),'--', label="$P(x)={0:.2}x^2 + {1:.2} x + {2:.2}$".format(*p))
plt.plot(x,y,'ok', alpha=0.7,label='Datos')
plt.legend(loc='best');
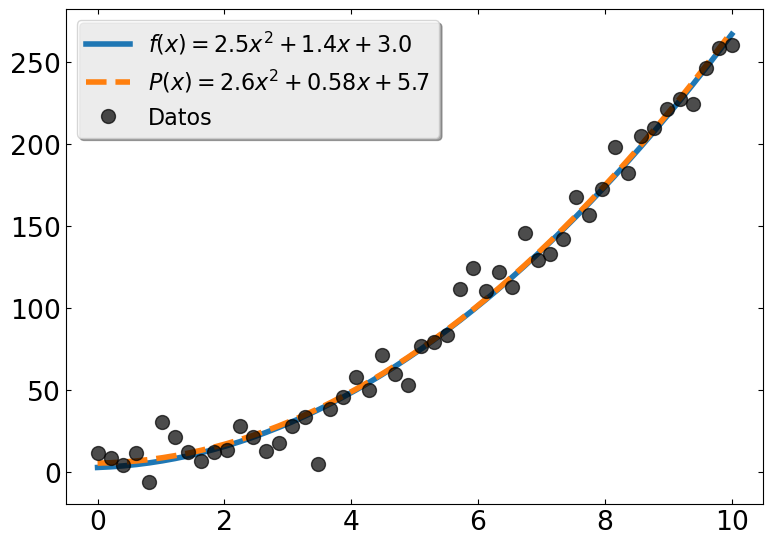
Ejercicios 13 (b)
En el archivo co_nrg.dat se encuentran los datos de la posición de los máximos de un espectro de CO2 como función del número cuántico rotacional J (entero). Haga un programa que lea los datos. Los ajuste con polinomios (elija el orden adecuado) y grafique luego los datos (con símbolos) y el ajuste con una línea sólida roja. Además, debe mostrar los parámetros obtenidos para el polinomio.
Fiteos con funciones arbitrarias
optimize
del paquete scipy
tiene rutinas para
realizar ajustes de datos utilizando funciones arbitrariasUtilicemos una función “complicada”:
# string definido para la leyenda
sfuncion= r'${0:.3}\, \sin ({1:.2}\, x {3:+.2f}) \, \exp(-{2:.2f} x)$'
def fit_func(x, a, b, c, d):
y = a*np.sin(b*x-d)*np.exp(-c*x)
return y
Generamos ahora “datos” con dispersión basados en la función
sfuncion.format(1.,2.,3.,4.)
x = np.linspace(0., 2*np.pi, 50)
y0 = fit_func(x, 1., 3., 1/3, 0 )
y = y0 + 0.1*ruido
y los graficamos
plt.figure(figsize=fsize)
plt.plot(x,y0,'-',label="$f(x)$")
plt.plot(x,y,'ok',alpha=0.5,label='Datos') # repeated from above
plt.xlabel("Eje x") # labels again
plt.ylabel("Eje y")
plt.legend(loc='best');
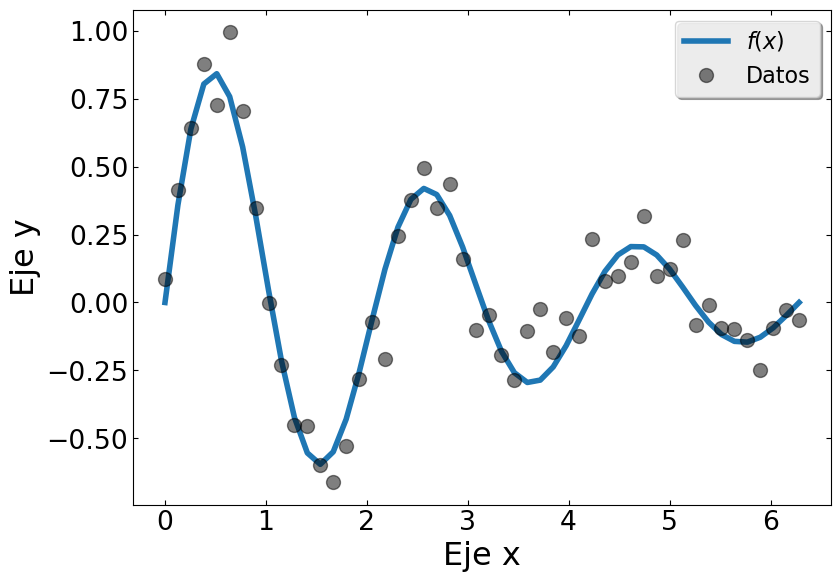
Ahora vamos a interpolar los datos utilizando funciones del paquete
Scipy. En primer lugar vamos a utilizar la función curve_fit
:
from scipy.optimize import curve_fit
# ¿Qué hace esta función?
help(curve_fit)
Help on function curve_fit in module scipy.optimize._minpack_py: curve_fit( f, xdata, ydata, p0=None, sigma=None, absolute_sigma=False, check_finite=None, bounds=(-inf, inf), method=None, jac=None, , full_output=False, nan_policy=None, **kwargs ) Use non-linear least squares to fit a function, f, to data. Assumes ``ydata = f(xdata, *params) + eps``. Parameters ---------- f : callable The model function, f(x, ...). It must take the independent variable as the first argument and the parameters to fit as separate remaining arguments. xdata : array_like The independent variable where the data is measured. Should usually be an M-length sequence or an (k,M)-shaped array for functions with k predictors, and each element should be float convertible if it is an array like object. ydata : array_like The dependent data, a length M array - nominally ``f(xdata, ...)``. p0 : array_like, optional Initial guess for the parameters (length N). If None, then the initial values will all be 1 (if the number of parameters for the function can be determined using introspection, otherwise a ValueError is raised). sigma : None or M-length sequence or MxM array, optional Determines the uncertainty in `ydata`. If we define residuals as ``r = ydata - f(xdata, *popt)``, then the interpretation of `sigma` depends on its number of dimensions: - A 1-D `sigma` should contain values of standard deviations of errors in `ydata`. In this case, the optimized function is ``chisq = sum((r / sigma) * 2)``. - A 2-D sigma should contain the covariance matrix of errors in ydata. In this case, the optimized function ischisq = r.T @ inv(sigma) @ r
. .. versionadded:: 0.19 None (default) is equivalent of 1-D sigma filled with ones. absolute_sigma : bool, optional If True, sigma is used in an absolute sense and the estimated parameter covariance pcov reflects these absolute values. If False (default), only the relative magnitudes of the sigma values matter. The returned parameter covariance matrix pcov is based on scaling sigma by a constant factor. This constant is set by demanding that the reduced chisq for the optimal parameters popt when using the scaled sigma equals unity. In other words, sigma is scaled to match the sample variance of the residuals after the fit. Default is False. Mathematically,pcov(absolute_sigma=False) = pcov(absolute_sigma=True) * chisq(popt)/(M-N)
check_finite : bool, optional If True, check that the input arrays do not contain nans of infs, and raise a ValueError if they do. Setting this parameter to False may silently produce nonsensical results if the input arrays do contain nans. Default is True if nan_policy is not specified explicitly and False otherwise. bounds : 2-tuple of array_like or Bounds, optional Lower and upper bounds on parameters. Defaults to no bounds. There are two ways to specify the bounds: - Instance of Bounds class. - 2-tuple of array_like: Each element of the tuple must be either an array with the length equal to the number of parameters, or a scalar (in which case the bound is taken to be the same for all parameters). Usenp.inf
with an appropriate sign to disable bounds on all or some parameters. method : {'lm', 'trf', 'dogbox'}, optional Method to use for optimization. See least_squares for more details. Default is 'lm' for unconstrained problems and 'trf' if bounds are provided. The method 'lm' won't work when the number of observations is less than the number of variables, use 'trf' or 'dogbox' in this case. .. versionadded:: 0.17 jac : callable, string or None, optional Function with signaturejac(x, ...)
which computes the Jacobian matrix of the model function with respect to parameters as a dense array_like structure. It will be scaled according to provided sigma. If None (default), the Jacobian will be estimated numerically. String keywords for 'trf' and 'dogbox' methods can be used to select a finite difference scheme, see least_squares. .. versionadded:: 0.18 full_output : boolean, optional If True, this function returns additioal information: infodict, mesg, and ier. .. versionadded:: 1.9 nan_policy : {'raise', 'omit', None}, optional Defines how to handle when input contains nan. The following options are available (default is None): * 'raise': throws an error * 'omit': performs the calculations ignoring nan values * None: no special handling of NaNs is performed (except what is done by check_finite); the behavior when NaNs are present is implementation-dependent and may change. Note that if this value is specified explicitly (not None), check_finite will be set as False. .. versionadded:: 1.11 **kwargs Keyword arguments passed to leastsq formethod='lm'
or least_squares otherwise. Returns ------- popt : array Optimal values for the parameters so that the sum of the squared residuals off(xdata, *popt) - ydata
is minimized. pcov : 2-D array The estimated approximate covariance of popt. The diagonals provide the variance of the parameter estimate. To compute one standard deviation errors on the parameters, useperr = np.sqrt(np.diag(pcov))
. Note that the relationship between cov and parameter error estimates is derived based on a linear approximation to the model function around the optimum [1]. When this approximation becomes inaccurate, cov may not provide an accurate measure of uncertainty. How the sigma parameter affects the estimated covariance depends on absolute_sigma argument, as described above. If the Jacobian matrix at the solution doesn't have a full rank, then 'lm' method returns a matrix filled withnp.inf
, on the other hand 'trf' and 'dogbox' methods use Moore-Penrose pseudoinverse to compute the covariance matrix. Covariance matrices with large condition numbers (e.g. computed with numpy.linalg.cond) may indicate that results are unreliable. infodict : dict (returned only if full_output is True) a dictionary of optional outputs with the keys:nfev
The number of function calls. Methods 'trf' and 'dogbox' do not count function calls for numerical Jacobian approximation, as opposed to 'lm' method.fvec
The residual values evaluated at the solution, for a 1-D sigma this is(f(x, *popt) - ydata)/sigma
.fjac
A permutation of the R matrix of a QR factorization of the final approximate Jacobian matrix, stored column wise. Together with ipvt, the covariance of the estimate can be approximated. Method 'lm' only provides this information.ipvt
An integer array of length N which defines a permutation matrix, p, such that fjac*p = q*r, where r is upper triangular with diagonal elements of nonincreasing magnitude. Column j of p is column ipvt(j) of the identity matrix. Method 'lm' only provides this information.qtf
The vector (transpose(q) * fvec). Method 'lm' only provides this information. .. versionadded:: 1.9 mesg : str (returned only if full_output is True) A string message giving information about the solution. .. versionadded:: 1.9 ier : int (returnned only if full_output is True) An integer flag. If it is equal to 1, 2, 3 or 4, the solution was found. Otherwise, the solution was not found. In either case, the optional output variable mesg gives more information. .. versionadded:: 1.9 Raises ------ ValueError if either ydata or xdata contain NaNs, or if incompatible options are used. RuntimeError if the least-squares minimization fails. OptimizeWarning if covariance of the parameters can not be estimated. See Also -------- least_squares : Minimize the sum of squares of nonlinear functions. scipy.stats.linregress : Calculate a linear least squares regression for two sets of measurements. Notes ----- Users should ensure that inputs xdata, ydata, and the output of f arefloat64
, or else the optimization may return incorrect results. Withmethod='lm'
, the algorithm uses the Levenberg-Marquardt algorithm through leastsq. Note that this algorithm can only deal with unconstrained problems. Box constraints can be handled by methods 'trf' and 'dogbox'. Refer to the docstring of least_squares for more information. References ---------- [1] K. Vugrin et al. Confidence region estimation techniques for nonlinear regression in groundwater flow: Three case studies. Water Resources Research, Vol. 43, W03423, :doi:`10.1029/2005WR004804` Examples -------- >>> import numpy as np >>> import matplotlib.pyplot as plt >>> from scipy.optimize import curve_fit >>> def func(x, a, b, c): ... return a * np.exp(-b * x) + c Define the data to be fit with some noise: >>> xdata = np.linspace(0, 4, 50) >>> y = func(xdata, 2.5, 1.3, 0.5) >>> rng = np.random.default_rng() >>> y_noise = 0.2 * rng.normal(size=xdata.size) >>> ydata = y + y_noise >>> plt.plot(xdata, ydata, 'b-', label='data') Fit for the parameters a, b, c of the function func: >>> popt, pcov = curve_fit(func, xdata, ydata) >>> popt array([2.56274217, 1.37268521, 0.47427475]) >>> plt.plot(xdata, func(xdata, *popt), 'r-', ... label='fit: a=%5.3f, b=%5.3f, c=%5.3f' % tuple(popt)) Constrain the optimization to the region of0 <= a <= 3
,0 <= b <= 1
and0 <= c <= 0.5
: >>> popt, pcov = curve_fit(func, xdata, ydata, bounds=(0, [3., 1., 0.5])) >>> popt array([2.43736712, 1. , 0.34463856]) >>> plt.plot(xdata, func(xdata, *popt), 'g--', ... label='fit: a=%5.3f, b=%5.3f, c=%5.3f' % tuple(popt)) >>> plt.xlabel('x') >>> plt.ylabel('y') >>> plt.legend() >>> plt.show() For reliable results, the model func should not be overparametrized; redundant parameters can cause unreliable covariance matrices and, in some cases, poorer quality fits. As a quick check of whether the model may be overparameterized, calculate the condition number of the covariance matrix: >>> np.linalg.cond(pcov) 34.571092161547405 # may vary The value is small, so it does not raise much concern. If, however, we were to add a fourth parameterd
to func with the same effect asa
: >>> def func(x, a, b, c, d): ... return a * d * np.exp(-b * x) + c # a and d are redundant >>> popt, pcov = curve_fit(func, xdata, ydata) >>> np.linalg.cond(pcov) 1.13250718925596e+32 # may vary Such a large value is cause for concern. The diagonal elements of the covariance matrix, which is related to uncertainty of the fit, gives more information: >>> np.diag(pcov) array([1.48814742e+29, 3.78596560e-02, 5.39253738e-03, 2.76417220e+28]) # may vary Note that the first and last terms are much larger than the other elements, suggesting that the optimal values of these parameters are ambiguous and that only one of these parameters is needed in the model.
En su forma más simple toma los siguientes argumentos:
Parameters
----------
f : callable
The model function, f(x, ...). It must take the independent
variable as the first argument and the parameters to fit as
separate remaining arguments.
xdata : An M-length sequence or an (k,M)-shaped array for functions with k predictors
The independent variable where the data is measured.
ydata : M-length sequence
The dependent data --- nominally f(xdata, ...)
p0 : None, scalar, or N-length sequence, optional
Initial guess for the parameters. If None, then the initial
values will all be 1 (if the number of parameters for the function
can be determined using introspection, otherwise a ValueError
is raised).
f
es la función que utilizamos para modelar los datos,
y que dependerá de la variable independiente x
y de los parámetros
a ajustar.Veamos lo que devuelve:
Returns
-------
popt : array
Optimal values for the parameters so that the sum of the squared error
of ``f(xdata, *popt) - ydata`` is minimized
pcov : 2d array
The estimated covariance of popt. The diagonals provide the variance
of the parameter estimate.
El primer array tiene los parámetros para “best-fit”, y el segundo da la estimación del error: la matriz de covarianza
Ya tenemos los valores a ajustar guardados en arrays x
e y
# initial_guess= None
initial_guess= [1., -1., 1., 0.2]
params, p_covarianza = curve_fit(fit_func, x, y, initial_guess)
plt.figure(figsize=(8,8))
plt.plot(x,y0,'-', alpha=0.7,label="$f(x)$")
plt.plot(x,y,'ok', alpha=0.5, label='Datos') # repeated from above
label=sfuncion.format(*params)
plt.plot(x,fit_func(x, *params), '--', label=label)
plt.xlabel("Eje x") # labels again
plt.ylabel("Eje y")
plt.legend(loc='best');
ylim = plt.ylim()
plt.ylim((ylim[0],1.2*ylim[1]));
Veamos otro ejemplo similar, con muchos datos y dispersión
from scipy.optimize import least_squares
# Puntos "experimentales" con dispersión
x = np.linspace(0., 2*np.pi, 5000)
y0= fit_func(x, 1., 3., 1/3, 0 )
y = y0 + 0.2* np.random.normal(loc= 0., scale= 1, size= y0.size);
# Fiteo
plt.figure(figsize=fsize)
plt.plot(x,y,'.k',label='datos') # repeated from above
[<matplotlib.lines.Line2D at 0x7fa88053c050>]
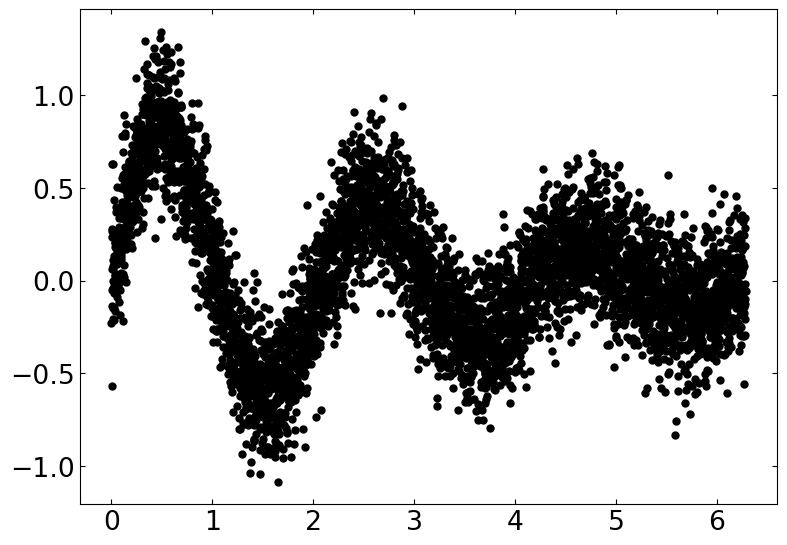
initial_guess= [.8, 3.5, 1., 0.2]
params, p_covarianza = curve_fit(fit_func, x, y, initial_guess)
# Graficación
plt.figure(figsize=fsize)
plt.plot(x,y,'.k',label='datos') # repeated from above
label=sfuncion.format(*params)
plt.plot(x,fit_func(x, *params), '-r', label=label)
plt.xlabel("Eje x") # labels again
plt.ylabel("Eje y")
plt.legend(loc='best');
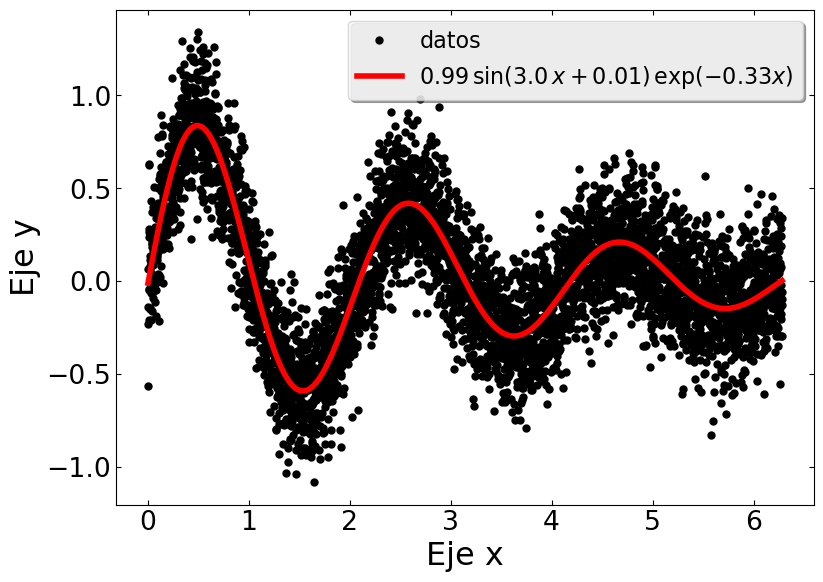
La función curve_fit()
que realiza el ajuste devuelve dos valores:
El primero es un array con los valores de los parámetros obtenidos, y
el segundo es un array con los valores correspondientes a la matriz de
covarianza, cuyos elementos de la diagonal corresponden a la varianza
para cada parámetro
params
array([0.98980641, 3.00530468, 0.33122372, 0.01168402])
p_covarianza.shape
(4, 4)
np.diagonal(p_covarianza)
array([1.65259123e-04, 4.03730946e-05, 4.45547667e-05, 1.48478575e-04])
Así, por ejemplo el primer parámetro (correspondiente a la amplitud
a
) toma en estos casos el valor:
for j,v in enumerate(['a','b', 'c', 'd' ]):
print("{} = {:.5g} ± {:.3g}".format(v, params[j], np.sqrt(p_covarianza[j,j])))
a = 0.98981 ± 0.0129
b = 3.0053 ± 0.00635
c = 0.33122 ± 0.00667
d = 0.011684 ± 0.0122
Ejemplo: Fiteo de picos
Vamos a suponer que los datos son obtenidos mediante la repetición de mediciones
# Realizamos 1000 mediciones, eso nos da una distribución de valores
r = np.random.normal(loc=6, size=1000)
# Veamos qué obtuvimos
plt.plot(r,'.')
[<matplotlib.lines.Line2D at 0x7fa880564410>]
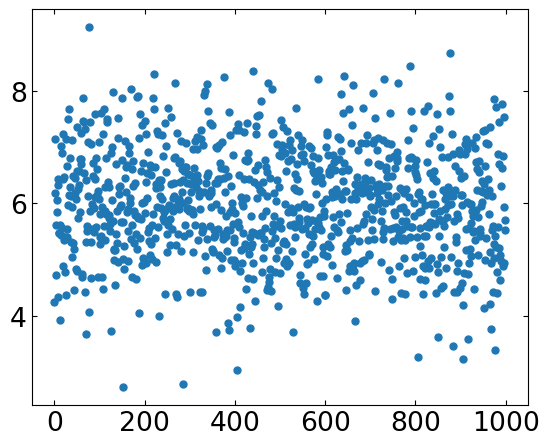
y, x = np.histogram(r, bins=30, range=(2,10), density=True)
x = (x[1:]+x[:-1])/2 # Calculamos los centros de los intervalos
Vamos a graficar el histograma junto con la función Gaussiana con el valor correspondiente de la “Función Densidad de Probabilidad” (pdf)
from scipy import stats
b = stats.norm.pdf(x, loc=6)
plt.figure(figsize=fsize)
plt.plot(x,b,'-', label=u'Función')
plt.plot(x,y,'o-', alpha=0.7, label='random')
plt.legend(loc='best');
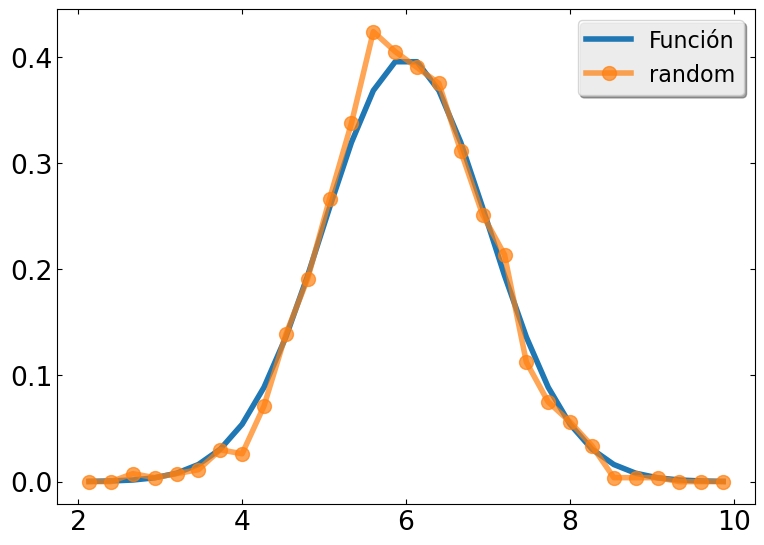
Usando esta idea generemos “datos” correspondiente a dos picos, que son diferentes y están separados pero que no podemos distinguirlos completamente porque se superponen.
Generemos los datos:
npoints = 3000
r = np.hstack([np.random.normal(size=npoints), np.random.normal(loc=2, scale=.6, size=npoints)])
y,x = np.histogram(r , bins = 40, range = (-3.5,4.5), density=True)
x = (x[1:]+x[:-1])/2
r.shape, r.size
((6000,), 6000)
plt.figure(figsize=fsize)
plt.plot(x,y,'o--k', alpha=0.6, label='"Medición"')
plt.legend(loc='best');
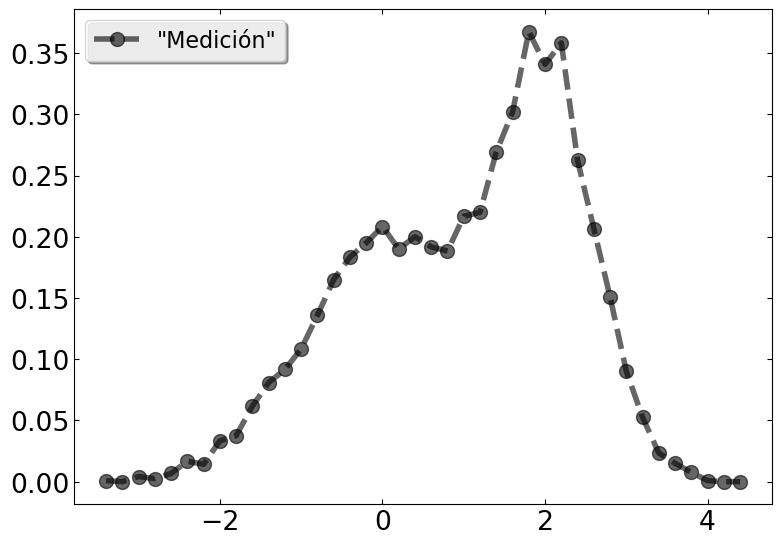
Ahora, por razones físicas (o porque no tenemos ninguna idea mejor) suponemos que esta curva corresponde a dos “picos” del tipo Gaussiano, sólo que no conocemos sus posiciones, ni sus anchos ni sus alturas relativas. Creamos entonces una función que describa esta situación: La suma de dos Gaussianas, cada una de ellas con su posición y ancho característico, y un factor de normalización diferente para cada una de ellas. En total tendremos seis parámetros a optimizar:
def modelo(x, *coeffs):
"Suma de dos Gaussianas, con pesos dados por coeffs[0] y coeffs[3], respectivamente"
G = stats.norm.pdf
return coeffs[0]*G(x,loc=coeffs[1], scale=coeffs[2]) + \
coeffs[3]*G(x,loc=coeffs[4], scale=coeffs[5])
help(modelo)
Help on function modelo in module __main__:
modelo(x, *coeffs)
Suma de dos Gaussianas, con pesos dados por coeffs[0] y coeffs[3], respectivamente
Ahora es muy fácil realizar el fiteo. Mirando el gráfico proponemos valores iniciales, donde los tres primeros valores corresponde a los parámetros de la primera Gaussiana y los tres últimos a los de la segunda:
c0 = np.array([1., -0.5, 1., 1., 1.5, 1.])
c, cov = curve_fit(modelo, x, y, p0 = c0)
print(c)
[0.52481498 0.07615262 1.04841506 0.47277331 2.03551126 0.5881539 ]
plt.figure(figsize=fsize)
plt.plot(x, y,'ok', alpha=0.8, label='random distrib')
plt.plot(x, modelo(x,*c0), '--', alpha=0.9, label='Curva inicial')
plt.plot(x, modelo(x,*c), '-', label='fiteo')
plt.plot(x,c[0]*stats.norm.pdf(x,loc=c[1], scale=c[2]), '--', alpha=0.7, label='Gaussiana 1')
plt.plot(x,c[3]*stats.norm.pdf(x,loc=c[4], scale=c[5]), '--', alpha=0.7, label='Gaussiana 2')
plt.legend( loc = 'best' );
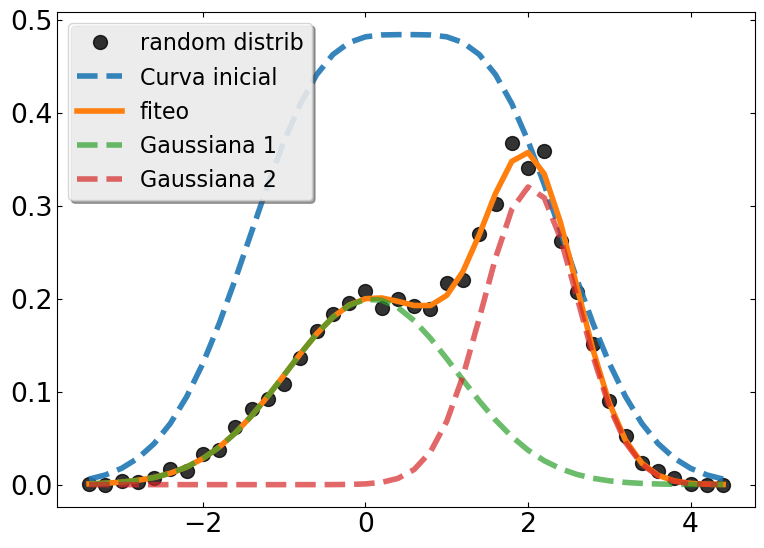
def modelo2(x, A1, x1, s1, A2, x2, s2):
"Suma de dos Gaussianas, con pesos dados por coeffs[0] y coeffs[3], respectivamente"
G = stats.norm.pdf
return A1*G(x,loc=x1, scale=s1) + \
A2*G(x,loc=x2, scale=s2)
c, cov = curve_fit(modelo2, x, y)
print(c)
[0.52481442 0.07615151 1.04841391 0.47277388 2.03551105 0.58815438]
plt.figure(figsize=fsize)
plt.plot(x, y,'ok', alpha=0.8, label='random distrib')
plt.plot(x, modelo2(x,*c), '-', label='fiteo')
plt.plot(x,c[0]*stats.norm.pdf(x,loc=c[1], scale=c[2]), '--', alpha=0.7, label='Gaussiana 1')
plt.plot(x,c[3]*stats.norm.pdf(x,loc=c[4], scale=c[5]), '--', alpha=0.7, label='Gaussiana 2')
plt.legend( loc = 'best' );
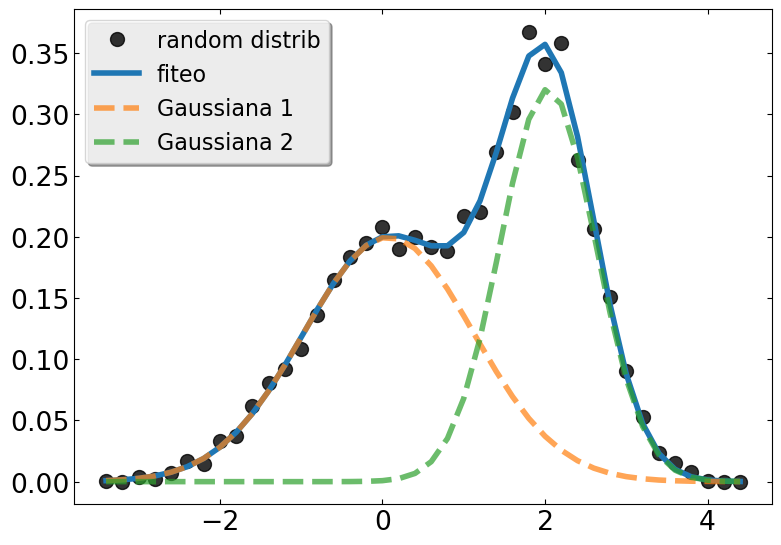
Ejercicios 13 (c)
Para Entregar: Queremos hacer un programa que permita fitear una curva como suma de
N
funciones gaussianas:Usando distribuciones normales e histogramas cree conjuntos de datos con dos, tres y cuatro máximos.
Haga una función, que debe tomar como argumento los arrays con los datos:
x
,y
, y valores iniciales para las Gaussianas:fit_gaussianas(x, y, *params)
donde params son los 3N coeficientes (tres coeficientes para cada Gaussiana). Debe devolver los parámetros óptimos obtenidos.Realice un programita que grafique los datos dados y la función que resulta de sumar las gaussianas en una misma figura.
Si puede agregue líneas o flechas indicando la posición del máximo y el ancho de cada una de las Gaussianas obtenidas.
Trabajo simple con imágenes
Vamos a empezar leyendo y mostrando algunas imágenes, para entender cómo
es su representación. Existen numerosos módulos que permiten manejar
imágenes, desde el ubicuo matplotlib
, pasando por PIL
(conda install pillow
) hasta openCV
(conda install opencv-python
).
import numpy as np
%matplotlib inline
import matplotlib.pyplot as plt
from PIL import Image
El siguiente ejemplo es una figura tomada de algunas pruebas que hicimos en el laboratorio hace unos años. Es el resultado de la medición de flujo en toberas
imag1 = plt.imread('figuras/imagen_flujo.jpg')
print(f'La imagen "imag1" es del tipo: {type(imag1)} con "shape" {imag1.shape}')
plt.imshow(imag1);
La imagen "imag1" es del tipo: <class 'numpy.ndarray'> con "shape" (272, 652, 3)
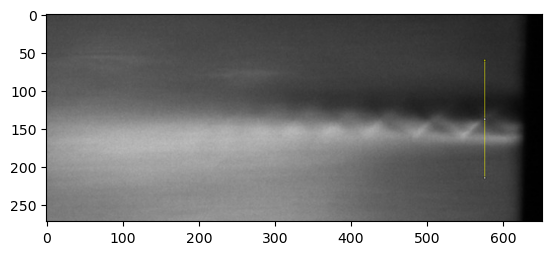
La representación de la imagen es una matriz, donde cada elemento
corresponde a un pixel, y cada pixel tiene tres valores. El elemento
[0,0]
corresponde al pixel ubicado en la esquina superior izquierda,
el elemento [-1,0]
al pixel ubicado en la esquina inferior
izquierda, mientras que el [0,-1]
a la esquina superior derecha:
plt.imshow(imag1)
color='white'
plt.annotate("[0,0]",(0,0), (60,40), arrowprops={}, fontsize='x-large', color=color)
plt.annotate("[-1,0]",(0,272), (60,240), arrowprops={}, fontsize='x-large', color=color)
plt.annotate("[0,-1]",(652,0), (510,40), arrowprops={}, fontsize='x-large', color=color);
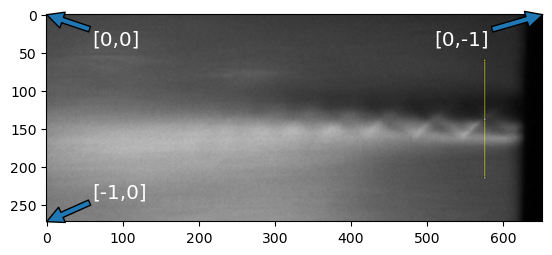
En consecuencia podemos ver qué valores toma cada pixel
print(imag1[0,0]) # El primer elemento
print(imag1[0,1]) # El segundo elemento
print(imag1.min(),imag1.max()) # y sus valores mínimo y máximo
[65 65 65]
[66 66 66]
0 255
Como vemos en cada pixel el valor está dado por un array de tres números enteros
imag1.dtype
dtype('uint8')
print(imag1[0,0]) # El primer elemento del primer pixel
[65 65 65]
Como originalmente teníamos una figura en escala de grises, podemos
convertir los tres colores a una simple escala, por ejemplo promediando
los tres valores. La función imread()
puede interpretar la figura
como una escala de grises con los argumentos adecuados:
Image.open('figuras/imagen_flujo.jpg').convert('L').save('figuras/imagen_flujo_gray.jpg')
imag2 = plt.imread('figuras/imagen_flujo_gray.jpg')
La variable imag2
contiene ahora una matriz con las dimensiones de
la imagen (272 x 652) pero con sólo un valor por cada pixel
print(imag2.shape)
print(imag2[0,0])
(272, 652)
65
plt.imshow(imag2);
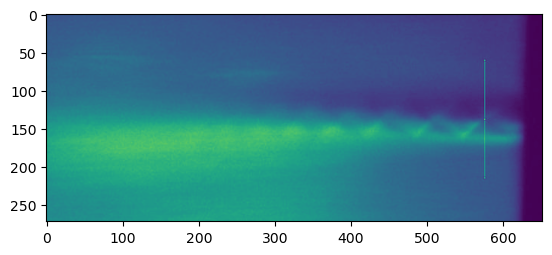
Nota ¿Qué pasó acá?
La función imshow()
está interpretando el valor de cada pixel como
una posición en una cierta escala de colores (colormap). Como no
especificamos cuál queremos utilizar, se usó el cmap default.
Especifiquemos el colormap a utilizar para la graficación:
plt.imshow(imag2, cmap='gray');
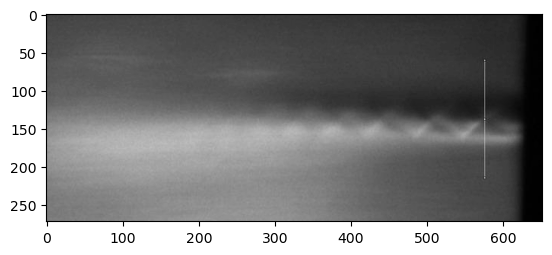
Los cmap
aparecen también en el contexto de graficación de gráficos
de contorno (contour()
y contourf()
).
Al asociar un valor a un mapa de colores, la misma imagen puede mostrarse de diferente maneras. Veamos otros ejemplos de colormap
plt.imshow(imag2, cmap='inferno');
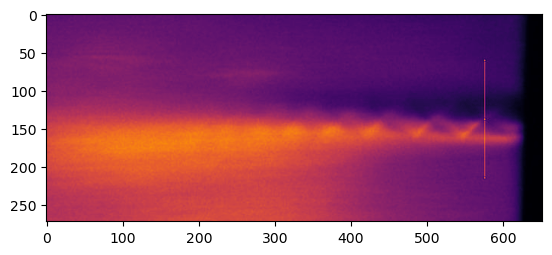
plt.imshow(imag2, cmap='inferno_r');
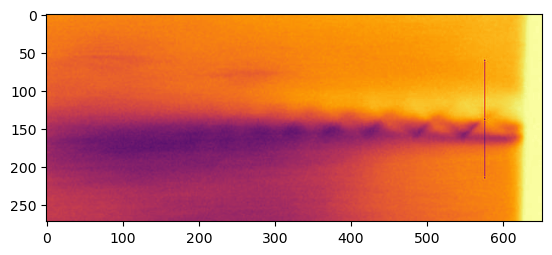
La referencia de ubicación de los cmap
existentes está en:
http://matplotlib.org/examples/color/colormaps_reference.html
plt.imshow(imag2, cmap='gray_r');
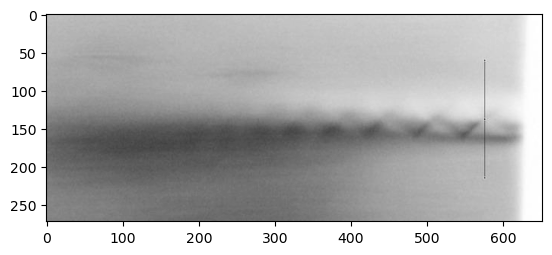
Análisis de la imagen
La imagen es una representación en mapa de colores de lo valores en la matriz. Esta es una representación que da muy buena información cualitativa sobre las características de los datos. Para analizar los datos a veces es más fácil hacer cortes o promediar en alguna dirección los datos.
Histograma de intensidades
Un ejemplo es el cálculo de un histograma de intensidades, analizando toda la imagen.
hist, bin_edges = np.histogram(imag2, bins=50, density=True)
bin_centers = 0.5*(bin_edges[:-1] + bin_edges[1:])
plt.fill_between(bin_centers, 0, hist, alpha=0.7)
plt.plot(bin_centers, hist, color='C0', lw=3)
plt.xlabel('Intensidad')
plt.xlim((0,200));
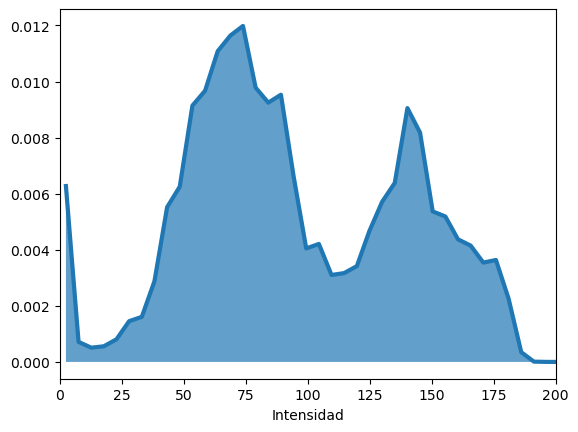
# Creamos una figura con los dos gráficos
fig, ax = plt.subplots(figsize=(10,2), ncols=2)
# En el gráfico de la izquierda mostramos la imagen en escala de grises
ax[0].imshow(imag2, cmap=plt.cm.gray, interpolation='nearest')
ax[0].axis('off') # Eliminamos los dos ejes
#
# Graficamos a la derecha el histograma
ax[1].plot(bin_centers, hist, lw=2)
ax[1].text(180, 0.85*hist.max(), 'Histograma', fontsize=20)
ax[1].set_yticks([]) # Sólo valores en el eje x
plt.subplots_adjust(wspace=-0.20, top=1, bottom=0.1, left=-0.2, right=1)
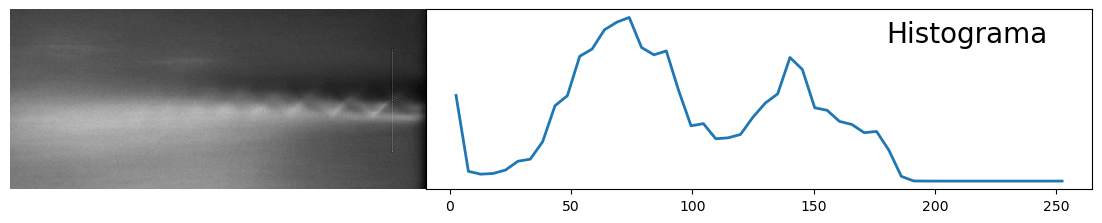
Estos histogramas, son útiles pero no dan información sobre las variaciones de intensidad con la posición. De alguna manera estamos integrando demasiado. Cuando vemos la imagen, vemos un mapa de intensidad en dos dimensiones. Al hacer el histograma sobre toda la figura perdemos completamente la información sobre la posición.
Cortes en una dirección
Un análisis intermedio podemos hacerlo haciendo cortes a lo largo de alguna línea y analizando la intensidad. Por ejemplo, podemos elegir una línea vertical en un punto \(x_0\), y analizar como varía la intensidad a lo largo de esa línea:
x0 = int(imag2.shape[1]*7/9) # Elegimos un punto en el eje x
print(f'posición en eje x={x0} de un total de {imag2.shape[1]}')
posición en eje x=507 de un total de 652
# Creamos la figura con dos subplots
fig, (ax2, ax1) = plt.subplots(ncols=2, figsize=(10,2))
# graficamos la imagen en el subplot de la derecha
ax1.imshow(imag2, cmap=plt.cm.gray)
# y agregamos la línea vertical en el punto elegido
ax1.axvline(x0, ls='--', lw=3)
ax1.text(1.05*x0, 50, '$x_{0}$', fontsize='x-large', color='C0')
ax1.axis('off')
#
# Creamos linea como un array 1D con los datos a lo largo de la línea deseada
# y la graficamos
linea = imag2[:,x0]
ax2.plot(linea,'--', lw=3, label='Corte')
ax2.set_xlabel(u'posición en eje $y$ (pixels)')
ax2.set_ylabel('Intensidad')
ax2.legend(loc='best')
ax2.set_xlim((0,len(linea)))
# Ajustamos el margen izquierdo y la distancia entre los dos subplots
plt.subplots_adjust(wspace=-0.1, left=0)
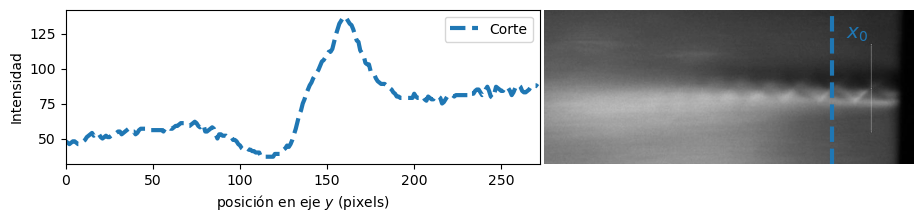
Trabajando con metadatos de la imagen
En la sección anterior vimos cómo trabajar con la imagen propiamente
dicha, que representa como un arreglo tridimensional de numpy
.
Además, es posible que la imagen contenga metadatos, esto es,
información asociada a la imagen que puede ser de utilidad.
type(imag2)
numpy.ndarray
img3 = Image.open('figuras/imagen_flujo.jpg')
type(img3)
PIL.JpegImagePlugin.JpegImageFile
metadata = img3.info
metadata
{'jfif': 257, 'jfif_version': (1, 1), 'jfif_unit': 2, 'jfif_density': (86, 86)}
Una de las ventajas del formato png es que soporta metadatos
arbitrarios, con lo cual podemos usar un plugin del modulo PIL
para
escribirlos:
from PIL.PngImagePlugin import PngInfo
new_metadata = PngInfo()
new_metadata.add_text("Autor", "F. Ulano")
new_metadata.add_text("Descripción", "Imagen de flujo en toberas")
# Agregamos la vieja metadata
for k,v in metadata.items():
new_metadata.add_itxt(k, str(v))
# new_metadata.add_text(k, str(v))
# Guardamos la imagen con la nueva metadata
img3.save('figuras/imagen_flujo_meta.png', pnginfo=new_metadata)
# Leemos la nueva imagen
img4 = Image.open('figuras/imagen_flujo_meta.png')
# Mostramos la metadata
img4.info
{'Autor': 'F. Ulano',
'Descripción': 'Imagen de flujo en toberas',
'jfif': '257',
'jfif_version': '(1, 1)',
'jfif_unit': '2',
'jfif_density': '(86, 86)'}
Otros formatos de imágenes (jpeg, por ejemplo) no soportan la
inclusión de metadata arbitraria. En cada caso será necesario
recurrir a módulos específicos para cada tipo de imagen.
En el caso de usar Image.open
de PIL
para leer el archivo de la
imagen, obtendremos la imagen propiamente dicha con
imagen = Image.open('figuras/imagen_flujo.jpg') # Cambiar por la ruta de la imagen
imagen_array = np.array(imagen)
print(imagen_array.shape) # Imprime las dimensiones de la imagen
(272, 652, 3)
Ejercicios 13 (d)
Modificar el ejemplo anterior para presentar en una figura tres gráficos, agregando a la izquierda un panel donde se muestre un corte horizontal. El corte debe estar en la mitad del gráfico (\(y_{0}=136\)). En la figura debe mostrar la posición del corte (similarmente a como se hizo con el corte en \(x\)) con una línea de otro color.
Dada una imagen en color (por ejemplo,
figuras/babbage.png
), escribe un programa que calcule y grafique los histogramas de los tres canales de color (rojo, verde y azul) en un único gráfico. Cada canal debe representarse con su color correspondiente.